Imagine you’re a coach preparing your team for a big competition. You’ve trained your players extensively, but there’s a catch—the game is going to be played on different fields with varying conditions. This is similar to the challenge faced by machine learning models in brain MRI segmentation. Although these models are highly effective, they often struggle when encountering variations in MRI data due to differences in scanner hardware, software, and acquisition parameters. That’s where domain adaptation (DA) techniques come in. They help the models adapt to these changes and perform consistently across different domains. In this study, researchers propose a benchmark that assesses the effectiveness of various DA techniques in brain MR image segmentation. They provide labeled data, source code, and a comprehensive evaluation framework for skull-stripping and segmenting different brain structures like white matter, gray matter, and cerebrospinal fluid. What’s fascinating is that no single DA technique emerged as the clear winner. The authors also highlight the ongoing challenges of hyperparameter tuning and computational efficiency, crucial factors for their integration into clinical practice. If you’re curious about the latest advancements in brain MRI segmentation and how different DA techniques measure up, dive into the full research article!
Accurate brain segmentation is critical for magnetic resonance imaging (MRI) analysis pipelines. Machine-learning-based brain MR image segmentation methods are among the state-of-the-art techniques for this task. Nevertheless, the segmentations produced by machine learning models often degrade in the presence of expected domain shifts between the test and train sets data distributions. These domain shifts are expected due to several factors, such as scanner hardware and software differences, technology updates, and differences in MRI acquisition parameters. Domain adaptation (DA) methods can make machine learning models more resilient to these domain shifts. This paper proposes a benchmark for investigating DA techniques for brain MR image segmentation using data collected across sites with scanners from different vendors (Philips, Siemens, and General Electric). Our work provides labeled data, publicly available source code for a set of baseline and DA models, and a benchmark for assessing different brain MR image segmentation techniques. We applied the proposed benchmark to evaluate two segmentation tasks: skull-stripping; and white-matter, gray-matter, and cerebrospinal fluid segmentation, but the benchmark can be extended to other brain structures. Our main findings during the development of this benchmark are that there is not a single DA technique that consistently outperforms others, and hyperparameter tuning and computational times for these methods still pose a challenge before broader adoption of these methods in the clinical practice.
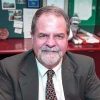
Dr. David Lowemann, M.Sc, Ph.D., is a co-founder of the Institute for the Future of Human Potential, where he leads the charge in pioneering Self-Enhancement Science for the Success of Society. With a keen interest in exploring the untapped potential of the human mind, Dr. Lowemann has dedicated his career to pushing the boundaries of human capabilities and understanding.
Armed with a Master of Science degree and a Ph.D. in his field, Dr. Lowemann has consistently been at the forefront of research and innovation, delving into ways to optimize human performance, cognition, and overall well-being. His work at the Institute revolves around a profound commitment to harnessing cutting-edge science and technology to help individuals lead more fulfilling and intelligent lives.
Dr. Lowemann’s influence extends to the educational platform BetterSmarter.me, where he shares his insights, findings, and personal development strategies with a broader audience. His ongoing mission is shaping the way we perceive and leverage the vast capacities of the human mind, offering invaluable contributions to society’s overall success and collective well-being.