Stroke rehabilitation is like climbing a mountain. With the increasing number of stroke patients, there is a pressing need for an accessible, scientific, and reliable evaluation method to guide their recovery journey. Just as climbers rely on GPS trackers to navigate their way up, researchers have developed a method using wearable sensors to evaluate the Brunnstrom recovery stage of the lower limb (BRS-L). In this study, motion and pressure data were collected from stroke patients and healthy individuals using special sensors. By analyzing these data, researchers were able to extract important gait features and improve the interpretability of the machine learning-based evaluation method. Interestingly, the results showed that the k-Nearest Neighbor model had the best prediction accuracy of 94.2% when fed with 18 carefully selected features. This exciting finding suggests that wearable sensor technology holds great promise for providing precise rehabilitation and enabling home-based care for hemiplegic patients. To learn more about this research and delve into the details, check out the full article!
With the increasing number of stroke patients, there is an urgent need for an accessible, scientific, and reliable evaluation method for stroke rehabilitation. Although many rehabilitation stage evaluation methods based on the wearable sensors and machine learning algorithm have been developed, the interpretable evaluation of the Brunnstrom recovery stage of the lower limb (BRS-L) is still lacking. The paper propose an interpretable BRS-L evaluation method based on wearable sensors. We collected lower limb motion data and plantar pressure data of 20 hemiplegic patients and 10 healthy individuals using seven Inertial Measurement Units (IMUs) and two plantar pressure insoles. Then we extracted gait features from the motion data and pressure data. By using feature selection based on feature importance, we improved the interpretability of the machine learning-based evaluation method. Several machine learning models are evaluated on the dataset, the results show that k-Nearest Neighbor has the best prediction performance and achieves 94.2% accuracy with an input of 18 features. Our method provides a feasible solution for precise rehabilitation and home-based rehabilitation of hemiplegic patients.
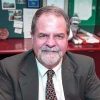
Dr. David Lowemann, M.Sc, Ph.D., is a co-founder of the Institute for the Future of Human Potential, where he leads the charge in pioneering Self-Enhancement Science for the Success of Society. With a keen interest in exploring the untapped potential of the human mind, Dr. Lowemann has dedicated his career to pushing the boundaries of human capabilities and understanding.
Armed with a Master of Science degree and a Ph.D. in his field, Dr. Lowemann has consistently been at the forefront of research and innovation, delving into ways to optimize human performance, cognition, and overall well-being. His work at the Institute revolves around a profound commitment to harnessing cutting-edge science and technology to help individuals lead more fulfilling and intelligent lives.
Dr. Lowemann’s influence extends to the educational platform BetterSmarter.me, where he shares his insights, findings, and personal development strategies with a broader audience. His ongoing mission is shaping the way we perceive and leverage the vast capacities of the human mind, offering invaluable contributions to society’s overall success and collective well-being.