Just as a detective uses clues to solve a mystery, scientists are using machine learning to uncover the secrets of dementia. Traditional diagnosis methods for dementia can be time-consuming and require lengthy questionnaires. To save time and reduce costs, researchers are developing a diagnosis model that uses machine learning to analyze questionnaire responses. They’re also using a feature selection method to pinpoint the most important questions, streamlining the process. By studying data from the Clinical Dementia Rating (CDR), scientists are able to categorize similar attributes and improve accuracy. The results of their experiments show that the bagging method is the most effective, with an impressive 80% accuracy rate. Additionally, principal component analysis (PCA) outperforms other feature selection methods. These findings demonstrate the power of machine learning in diagnosing dementia and could lead to more efficient and accurate assessments in the future.
As the aging population poses serious challenges to families and societies, the issue of dementia has also received increasing attention. Dementia detection often requires a series of complex tests and lengthy questionnaires, which are time-consuming. In order to solve this problem, this article aims at the diagnosis method of questionnaire survey, hoping to establish a diagnosis model to help doctors make a diagnosis through machine learning method, and use feature selection method to select important questions to reduce the number of questions in the questionnaire, so as to reduce medical and time costs. In this article, Clinical Dementia Rating (CDR) is used as the data source, and various methods are used for modeling and feature selection, so as to combine similar attributes in the data set, reduce the categories, and finally use the confusion matrix to judge the effect. The experimental results show that the model established by the bagging method has the best effect, and the accuracy rate can reach 80% of the true diagnosis rate; in terms of feature selection, the principal component analysis (PCA) has the best effect compared with other methods.
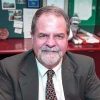
Dr. David Lowemann, M.Sc, Ph.D., is a co-founder of the Institute for the Future of Human Potential, where he leads the charge in pioneering Self-Enhancement Science for the Success of Society. With a keen interest in exploring the untapped potential of the human mind, Dr. Lowemann has dedicated his career to pushing the boundaries of human capabilities and understanding.
Armed with a Master of Science degree and a Ph.D. in his field, Dr. Lowemann has consistently been at the forefront of research and innovation, delving into ways to optimize human performance, cognition, and overall well-being. His work at the Institute revolves around a profound commitment to harnessing cutting-edge science and technology to help individuals lead more fulfilling and intelligent lives.
Dr. Lowemann’s influence extends to the educational platform BetterSmarter.me, where he shares his insights, findings, and personal development strategies with a broader audience. His ongoing mission is shaping the way we perceive and leverage the vast capacities of the human mind, offering invaluable contributions to society’s overall success and collective well-being.