Imagine you’re putting together a massive jigsaw puzzle, but some of the pieces are damaged. How do you make sure they still fit together seamlessly? That’s the challenge researchers face when reconstructing biological tissues using serial section electron microscopy (ssEM) images. Damaged sections can throw off the registration process, making it difficult to track and reconstruct neurons accurately. But fear not! A group of scientists has come up with a clever solution. They’ve developed a general registration method that can handle damaged sections with ease. By extracting key points and descriptors from the damaged sections and using advanced techniques like K-means clustering and Random Sample Consensus (RANSAC), they’re able to approximate the local affine matrices and find the best match for each coordinate point. To measure the correlation between sampling points, they even use the path distance instead of the traditional Euclidean distance. The results are impressive, showing that this method effectively registers damaged sections and contributes to the 3D reconstruction of biological tissue images. If you’re interested in learning more about this exciting research, check out the link below!
Registration is essential for the volume reconstruction of biological tissues using serial section electron microscope (ssEM) images. However, due to environmental disturbance in section preparation, damage in long serial sections is inevitable. It is difficult to register the damaged sections with the common serial section registration method, creating significant challenges in subsequent neuron tracking and reconstruction. This paper proposes a general registration method that can be used to register damaged sections. This method first extracts the key points and descriptors of the sections to be registered and matches them via a mutual nearest neighbor matcher. K-means and Random Sample Consensus (RANSAC) are used to cluster the key points and approximate the local affine matrices of those clusters. Then, K-nearest neighbor (KNN) is used to estimate the probability density of each cluster and calculate the expected affine matrix for each coordinate point. In clustering and probability density calculations, instead of the Euclidean distance, the path distance is used to measure the correlation between sampling points. The experimental results on real test images show that this method solves the problem of registering damaged sections and contributes to the 3D reconstruction of electronic microscopic images of biological tissues. The code of this paper is available at https://github.com/TongXin-CASIA/Excepted_Affine.
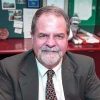
Dr. David Lowemann, M.Sc, Ph.D., is a co-founder of the Institute for the Future of Human Potential, where he leads the charge in pioneering Self-Enhancement Science for the Success of Society. With a keen interest in exploring the untapped potential of the human mind, Dr. Lowemann has dedicated his career to pushing the boundaries of human capabilities and understanding.
Armed with a Master of Science degree and a Ph.D. in his field, Dr. Lowemann has consistently been at the forefront of research and innovation, delving into ways to optimize human performance, cognition, and overall well-being. His work at the Institute revolves around a profound commitment to harnessing cutting-edge science and technology to help individuals lead more fulfilling and intelligent lives.
Dr. Lowemann’s influence extends to the educational platform BetterSmarter.me, where he shares his insights, findings, and personal development strategies with a broader audience. His ongoing mission is shaping the way we perceive and leverage the vast capacities of the human mind, offering invaluable contributions to society’s overall success and collective well-being.