Imagine your brain as a master learner, constantly acquiring new information while guarding the memories of past experiences. Scientists have developed a computational model inspired by the brain’s neural networks, specifically the hippocampus and its surrounding regions, to shed light on how this remarkable lifelong learning ability works. This model incorporates two key mechanisms: one involving dopamine, a neurotransmitter that enhances plasticity when triggered by novel stimuli, and another involving inhibitory connections among neurons in the hippocampus. These mechanisms work together to protect previously encoded memories from being disrupted when learning new information. By testing the model with various image datasets, researchers found that it effectively mitigates catastrophic interference, allowing for improved learning of new stimuli without erasing prior knowledge. This study opens up exciting possibilities for further exploration through animal experiments and may also inspire advancements in machine learning algorithms.
The human brain has a remarkable lifelong learning capability to acquire new experiences while retaining previously acquired information. Several hypotheses have been proposed to explain this capability, but the underlying mechanisms are still unclear. Here, we propose a neuro-inspired firing-rate computational model involving the hippocampus and surrounding areas, that encompasses two key mechanisms possibly underlying this capability. The first is based on signals encoded by the neuromodulator dopamine, which is released by novel stimuli and enhances plasticity only when needed. The second is based on a homeostatic plasticity mechanism that involves the lateral inhibitory connections of the pyramidal neurons of the hippocampus. These mechanisms tend to protect neurons that have already been heavily employed in encoding previous experiences. The model was tested with images from the MNIST machine learning dataset, and with more naturalistic images, for its ability to mitigate catastrophic interference in lifelong learning. The results show that the proposed biologically grounded mechanisms can effectively enhance the learning of new stimuli while protecting previously acquired knowledge. The proposed mechanisms could be investigated in future empirical animal experiments and inspire machine learning models.
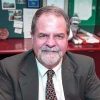
Dr. David Lowemann, M.Sc, Ph.D., is a co-founder of the Institute for the Future of Human Potential, where he leads the charge in pioneering Self-Enhancement Science for the Success of Society. With a keen interest in exploring the untapped potential of the human mind, Dr. Lowemann has dedicated his career to pushing the boundaries of human capabilities and understanding.
Armed with a Master of Science degree and a Ph.D. in his field, Dr. Lowemann has consistently been at the forefront of research and innovation, delving into ways to optimize human performance, cognition, and overall well-being. His work at the Institute revolves around a profound commitment to harnessing cutting-edge science and technology to help individuals lead more fulfilling and intelligent lives.
Dr. Lowemann’s influence extends to the educational platform BetterSmarter.me, where he shares his insights, findings, and personal development strategies with a broader audience. His ongoing mission is shaping the way we perceive and leverage the vast capacities of the human mind, offering invaluable contributions to society’s overall success and collective well-being.