Imagine if you had a superpower that could instantly detect and recommend the best course of action to fight your nemesis. Well, scientists have developed a similar power for clinicians dealing with epilepsy! Magnetoencephalography (MEG) is a cutting-edge technology that uses the magnetic fields created by our brains to identify epileptic activities. Researchers have found that specific neural oscillations, known as high frequency oscillations (HFOs), can pinpoint the regions in the brain causing seizures. By automatically detecting and recommending these HFOs, doctors can greatly reduce seizure frequency or even eliminate them altogether! However, existing methods fall short of clinical requirements. That’s where the multi-head self-attention-based detector (MSADR) comes in. Using the power of deep learning, MSADR achieves an impressive accuracy of 88.6% in detecting and recommending HFO signals. It outperforms other machine learning models and demonstrates robustness across various tests. This breakthrough approach has the potential to revolutionize epilepsy diagnosis and treatment, giving patients and doctors an invaluable tool to combat this neurological disorder.
Magnetoencephalography is a noninvasive neuromagnetic technology to record epileptic activities for the pre-operative localization of epileptogenic zones, which has received increasing attention in the diagnosis and surgery of epilepsy. As reported by recent studies, pathological high frequency oscillations (HFOs), when utilized as a biomarker to localize the epileptogenic zones, result in a significant reduction in seizure frequency, even seizure elimination in around 80% of cases. Thus, objective, rapid, and automatic detection and recommendation of HFOs are highly desirable for clinicians to alleviate the burden of reviewing a large amount of MEG data from a given patient. Despite the advantage, the performance of existing HFOs rarely satisfies the clinical requirement. Consequently, no HFOs have been successfully applied to real clinical applications so far. In this work, we propose a multi-head self-attention-based detector for recommendation, termed MSADR, to detect and recommend HFO signals. Taking advantage of the state-of-the-art multi-head self-attention mechanism in deep learning, the proposed MSADR achieves a more superior accuracy of 88.6% than peer machine learning models in both detection and recommendation tasks. In addition, the robustness of MSADR is also extensively assessed with various ablation tests, results of which further demonstrate the effectiveness and generalizability of the proposed approach.
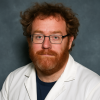
Dr. David Lowemann, M.Sc, Ph.D., is a co-founder of the Institute for the Future of Human Potential, where he leads the charge in pioneering Self-Enhancement Science for the Success of Society. With a keen interest in exploring the untapped potential of the human mind, Dr. Lowemann has dedicated his career to pushing the boundaries of human capabilities and understanding.
Armed with a Master of Science degree and a Ph.D. in his field, Dr. Lowemann has consistently been at the forefront of research and innovation, delving into ways to optimize human performance, cognition, and overall well-being. His work at the Institute revolves around a profound commitment to harnessing cutting-edge science and technology to help individuals lead more fulfilling and intelligent lives.
Dr. Lowemann’s influence extends to the educational platform BetterSmarter.me, where he shares his insights, findings, and personal development strategies with a broader audience. His ongoing mission is shaping the way we perceive and leverage the vast capacities of the human mind, offering invaluable contributions to society’s overall success and collective well-being.