Imagine having a crystal ball that could predict the risk of developing Parkinson’s disease (PD) or the progression of its non-motor symptoms. Well, researchers have come pretty close! In a recent study, scientists developed and tested predictive models using various factors to identify individuals at an early stage of PD and predict the progression of non-motor symptoms like cognitive impairment, Rapid-eye-movement sleep Behavior Disorder (RBD), and depression. By analyzing data from the Parkinson’s Progression Markers Initiative, they successfully constructed diagnostic models with an impressive accuracy rate of 93%! These models included factors such as age, gender, family history, smell inventory test score, cognitive assessment score, RBD screening questionnaire score, cerebrospinal fluid α-synuclein levels, and SNCA rs356181 polymorphism. Additionally, the prognostic models for non-motor symptom progression showed promising results. While the overall accuracy fluctuated around 70%, it improved with longer follow-up times. These findings provide valuable insights into predicting PD onset and non-motor symptom progression. Further research and validation are still needed to fully understand and extend the predictive capabilities of these models. Exciting times lie ahead in the field of PD research!
Unleashing the Crystal Ball: Predicting Parkinson’s Disease and Non-Motor Symptoms
BackgroundIdentifying individuals with high-risk Parkinson’s disease (PD) at earlier stages is an urgent priority to delay disease onset and progression. In the present study, we aimed to develop and validate clinical risk models using non-motor predictors to distinguish between early PD and healthy individuals. In addition, we constructed prognostic models for predicting the progression of non-motor symptoms [cognitive impairment, Rapid-eye-movement sleep Behavior Disorder (RBD), and depression] in de novo PD patients at 5 years of follow-up.MethodsWe retrieved the data from the Parkinson’s Progression Markers Initiative (PPMI) database. After a backward variable selection approach to identify predictors, logistic regression analyses were applied for diagnosis model construction, and cox proportional-hazards models were used to predict non-motor symptom progression. The predictive models were internally validated by correcting measures of predictive performance for “optimism” or overfitting with the bootstrap resampling approach.ResultsFor constructing diagnostic models, the final model reached a high accuracy with an area under the curve (AUC) of 0.93 (95% CI: 0.91–0.96), which included eight variables (age, gender, family history, University of Pennsylvania Smell Inventory Test score, Montreal Cognitive Assessment score, RBD Screening Questionnaire score, levels of cerebrospinal fluid α-synuclein, and SNCA rs356181 polymorphism). For the construction of prognostic models, our results showed that the AUC of the three prognostic models improved slightly with increasing follow-up time. The overall AUCs fluctuated around 0.70. The model validation established good discrimination and calibration for predicting PD onset and progression of non-motor symptoms.ConclusionThe findings of our study facilitate predicting the individual risk at an early stage based on the predictors derived from these models. These predictive models provide relatively reliable information to prevent PD onset and progression. However, future validation analysis is still needed to clarify these findings and provide more insight into the predictive models over more extended periods of disease progression in more diverse samples.
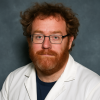
Dr. David Lowemann, M.Sc, Ph.D., is a co-founder of the Institute for the Future of Human Potential, where he leads the charge in pioneering Self-Enhancement Science for the Success of Society. With a keen interest in exploring the untapped potential of the human mind, Dr. Lowemann has dedicated his career to pushing the boundaries of human capabilities and understanding.
Armed with a Master of Science degree and a Ph.D. in his field, Dr. Lowemann has consistently been at the forefront of research and innovation, delving into ways to optimize human performance, cognition, and overall well-being. His work at the Institute revolves around a profound commitment to harnessing cutting-edge science and technology to help individuals lead more fulfilling and intelligent lives.
Dr. Lowemann’s influence extends to the educational platform BetterSmarter.me, where he shares his insights, findings, and personal development strategies with a broader audience. His ongoing mission is shaping the way we perceive and leverage the vast capacities of the human mind, offering invaluable contributions to society’s overall success and collective well-being.