Imagine a synchronized dance where dancers move in beautiful harmony, but instead of people, it’s inhibitory neurons in a 2D network. These networks often display synchronous assembly or clustered firing, where subsets of neurons fire together. Previous research focused on 1D or symmetric 2D networks, but this study looks at a general torus-shaped network with different coupling strengths in all directions. By using phase model analysis, the researchers determine the conditions for stability of different patterns of clustered firing behavior. They also explore how variations in network connectivity and heterogeneous coupling strengths affect which patterns are stable. The findings reveal that what works for a 1D network doesn’t necessarily apply to a 2D network, and that the diversity of coupling strengths plays a crucial role in determining stable patterns. If you want to delve into the intricacies of inhibitory neural networks and their mesmerizing synchronized dance, check out the full article!
Neural firing in many inhibitory networks displays synchronous assembly or clustered firing, in which subsets of neurons fire synchronously, and these subsets may vary with different inputs to, or states of, the network. Most prior analytical and computational modeling of such networks has focused on 1D networks or 2D networks with symmetry (often circular symmetry). Here, we consider a 2D discrete network model on a general torus, where neurons are coupled to two or more nearest neighbors in three directions (horizontal, vertical, and diagonal), and allow different coupling strengths in all directions. Using phase model analysis, we establish conditions for the stability of different patterns of clustered firing behavior in the network. We then apply our results to study how variation of network connectivity and the presence of heterogeneous coupling strengths influence which patterns are stable. We confirm and supplement our results with numerical simulations of biophysical inhibitory neural network models. Our work shows that 2D networks may exhibit clustered firing behavior that cannot be predicted as a simple generalization of a 1D network, and that heterogeneity of coupling can be an important factor in determining which patterns are stable.
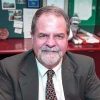
Dr. David Lowemann, M.Sc, Ph.D., is a co-founder of the Institute for the Future of Human Potential, where he leads the charge in pioneering Self-Enhancement Science for the Success of Society. With a keen interest in exploring the untapped potential of the human mind, Dr. Lowemann has dedicated his career to pushing the boundaries of human capabilities and understanding.
Armed with a Master of Science degree and a Ph.D. in his field, Dr. Lowemann has consistently been at the forefront of research and innovation, delving into ways to optimize human performance, cognition, and overall well-being. His work at the Institute revolves around a profound commitment to harnessing cutting-edge science and technology to help individuals lead more fulfilling and intelligent lives.
Dr. Lowemann’s influence extends to the educational platform BetterSmarter.me, where he shares his insights, findings, and personal development strategies with a broader audience. His ongoing mission is shaping the way we perceive and leverage the vast capacities of the human mind, offering invaluable contributions to society’s overall success and collective well-being.