Existing treatments can only delay the progression of spinocerebellar ataxia type 3/Machado-Joseph disease (SCA3/MJD) after onset, so the prediction of the age at onset (AAO) can facilitate early intervention and follow-up to improve treatment efficacy. The objective of this study was to develop an explainable artificial intelligence (XAI) based on feature optimization to provide an interpretable and more accurate AAO prediction. A total of 1,008 affected SCA3/MJD subjects from mainland China were analyzed. The expanded cytosine-adenine-guanine (CAG) trinucleotide repeats of 10 polyQ-related genes were genotyped and included in related models as potential AAO modifiers. The performance of 4 feature optimization methods and 10 machine learning (ML) algorithms were compared, followed by building the XAI based on the SHapley Additive exPlanations (SHAP). The model constructed with an artificial neural network (ANN) and feature optimization of Crossing-Correlation-StepSVM performed best and achieved a coefficient of determination (R2) of 0.653 and mean absolute error (MAE), root mean square error (RMSE), and median absolute error (MedianAE) of 4.544, 6.090, and 3.236 years, respectively. The XAI explained the predicted results, which suggests that the factors affecting the AAO were complex and associated with gene interactions. An XAI based on feature optimization can improve the accuracy of AAO prediction and provide interpretable and personalized prediction.
Read Full Article (External Site)
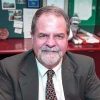
Dr. David Lowemann, M.Sc, Ph.D., is a co-founder of the Institute for the Future of Human Potential, where he leads the charge in pioneering Self-Enhancement Science for the Success of Society. With a keen interest in exploring the untapped potential of the human mind, Dr. Lowemann has dedicated his career to pushing the boundaries of human capabilities and understanding.
Armed with a Master of Science degree and a Ph.D. in his field, Dr. Lowemann has consistently been at the forefront of research and innovation, delving into ways to optimize human performance, cognition, and overall well-being. His work at the Institute revolves around a profound commitment to harnessing cutting-edge science and technology to help individuals lead more fulfilling and intelligent lives.
Dr. Lowemann’s influence extends to the educational platform BetterSmarter.me, where he shares his insights, findings, and personal development strategies with a broader audience. His ongoing mission is shaping the way we perceive and leverage the vast capacities of the human mind, offering invaluable contributions to society’s overall success and collective well-being.