Imagine you’re trying to watch your favorite show, but the picture is blurry and pixelated. Sure, you can make out what’s happening, but you’re missing all the important details. That’s how it feels when doctors use low-resolution Magnetic Resonance Images (MRI) for clinical diagnosis. These scans are like watching a show on a tiny old TV. They get the job done, but they’re not ideal for precision medicine or collaborative research. But fear not! Scientists have developed a groundbreaking method to enhance the quality of brain MRIs. They use a model that cleverly upscales low-resolution scans to create high-quality images. It’s like upgrading from that old TV to a crystal-clear HD screen! The model incorporates feature-importance and self-attention methods to improve the accuracy of the upscaled images. The results are remarkable, with the upsampled MRIs closely resembling the high-quality ground-truth scans. Moreover, the super-resolved images outperform interpolated images in accurately segmenting tissues and lesions. This exciting breakthrough opens up new possibilities for using MRIs in clinical practice and collaborative research!
Vast quantities of Magnetic Resonance Images (MRI) are routinely acquired in clinical practice but, to speed up acquisition, these scans are typically of a quality that is sufficient for clinical diagnosis but sub-optimal for large-scale precision medicine, computational diagnostics, and large-scale neuroimaging collaborative research. Here, we present a critic-guided framework to upsample low-resolution (often 2D) MRI full scans to help overcome these limitations. We incorporate feature-importance and self-attention methods into our model to improve the interpretability of this study. We evaluate our framework on paired low- and high-resolution brain MRI structural full scans (i.e., T1-, T2-weighted, and FLAIR sequences are simultaneously input) obtained in clinical and research settings from scanners manufactured by Siemens, Phillips, and GE. We show that the upsampled MRIs are qualitatively faithful to the ground-truth high-quality scans (PSNR = 35.39; MAE = 3.78E−3; NMSE = 4.32E−10; SSIM = 0.9852; mean normal-appearing gray/white matter ratio intensity differences ranging from 0.0363 to 0.0784 for FLAIR, from 0.0010 to 0.0138 for T1-weighted and from 0.0156 to 0.074 for T2-weighted sequences). The automatic raw segmentation of tissues and lesions using the super-resolved images has fewer false positives and higher accuracy than those obtained from interpolated images in protocols represented with more than three sets in the training sample, making our approach a strong candidate for practical application in clinical and collaborative research.
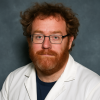
Dr. David Lowemann, M.Sc, Ph.D., is a co-founder of the Institute for the Future of Human Potential, where he leads the charge in pioneering Self-Enhancement Science for the Success of Society. With a keen interest in exploring the untapped potential of the human mind, Dr. Lowemann has dedicated his career to pushing the boundaries of human capabilities and understanding.
Armed with a Master of Science degree and a Ph.D. in his field, Dr. Lowemann has consistently been at the forefront of research and innovation, delving into ways to optimize human performance, cognition, and overall well-being. His work at the Institute revolves around a profound commitment to harnessing cutting-edge science and technology to help individuals lead more fulfilling and intelligent lives.
Dr. Lowemann’s influence extends to the educational platform BetterSmarter.me, where he shares his insights, findings, and personal development strategies with a broader audience. His ongoing mission is shaping the way we perceive and leverage the vast capacities of the human mind, offering invaluable contributions to society’s overall success and collective well-being.