Imagine you have a magical translator that can understand and interpret your emotions. That’s exactly what the E2ENNet neural network aims to do with the help of brain-computer interfaces. Just like deciphering a secret language, this innovative network can recognize and regulate human emotions. Instead of taking a step-by-step approach like traditional methods, E2ENNet streamlines the process by directly extracting features from raw EEG signals. It’s like having a direct line to your thoughts and feelings! With impressive accuracy rates on public datasets, E2ENNet proves its effectiveness in emotion recognition tasks. This breakthrough opens up exciting possibilities for workload detection and auxiliary diagnosis of mental illness. The paper provides detailed methodology and experimental results, giving researchers and developers the tools to implement their own emotional brain-computer interface systems. Get ready to unlock the power of your mind!
ObjectveEmotional brain-computer interface can recognize or regulate human emotions for workload detection and auxiliary diagnosis of mental illness. However, the existing EEG emotion recognition is carried out step by step in feature engineering and classification, resulting in high engineering complexity and limiting practical applications in traditional EEG emotion recognition tasks. We propose an end-to-end neural network, i.e., E2ENNet.MethodsBaseline removal and sliding window slice used for preprocessing of the raw EEG signal, convolution blocks extracted features, LSTM network obtained the correlations of features, and the softmax function classified emotions.ResultsExtensive experiments in subject-dependent experimental protocol are conducted to evaluate the performance of the proposed E2ENNet, achieves state-of-the-art accuracy on three public datasets, i.e., 96.28% of 2-category experiment on DEAP dataset, 98.1% of 2-category experiment on DREAMER dataset, and 41.73% of 7-category experiment on MPED dataset.ConclusionExperimental results show that E2ENNet can directly extract more discriminative features from raw EEG signals.SignificanceThis study provides a methodology for implementing a plug-and-play emotional brain-computer interface system.
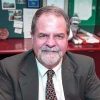
Dr. David Lowemann, M.Sc, Ph.D., is a co-founder of the Institute for the Future of Human Potential, where he leads the charge in pioneering Self-Enhancement Science for the Success of Society. With a keen interest in exploring the untapped potential of the human mind, Dr. Lowemann has dedicated his career to pushing the boundaries of human capabilities and understanding.
Armed with a Master of Science degree and a Ph.D. in his field, Dr. Lowemann has consistently been at the forefront of research and innovation, delving into ways to optimize human performance, cognition, and overall well-being. His work at the Institute revolves around a profound commitment to harnessing cutting-edge science and technology to help individuals lead more fulfilling and intelligent lives.
Dr. Lowemann’s influence extends to the educational platform BetterSmarter.me, where he shares his insights, findings, and personal development strategies with a broader audience. His ongoing mission is shaping the way we perceive and leverage the vast capacities of the human mind, offering invaluable contributions to society’s overall success and collective well-being.