Imagine trying to solve a complex puzzle with missing pieces. That’s what scientists face when trying to understand Alzheimer’s disease (AD). In this study, researchers used a combination of weighted gene co-expression network analysis (WGCNA) and machine learning to shed light on the pathological mechanism behind AD. They examined gene expression patterns in different brain regions with varying stages of AD progression. By analyzing the data, they discovered that these regions share similarities in their gene expression patterns, pointing to the universality of AD. The study also uncovered the crucial role of oxidative damage in the formation of neurofibrillary tangles (NFTs), which are the hallmark of AD and responsible for cognitive decline. Through sophisticated algorithms and statistical models, the researchers identified key genes involved in the development of AD, such as LYN, LAPTM5, and IFI30. These genes may be pivotal players in triggering an inflammatory response mediated by microglia, leading to AD progression. The findings from this study pave the way for further investigations into potential therapeutic targets for tackling this devastating disease.
Aberrant deposits of neurofibrillary tangles (NFT), the main characteristic of Alzheimer’s disease (AD), are highly related to cognitive impairment. However, the pathological mechanism of NFT formation is still unclear. This study explored differences in gene expression patterns in multiple brain regions [entorhinal, temporal, and frontal cortex (EC, TC, FC)] with distinct Braak stages (0- VI), and identified the hub genes via weighted gene co-expression network analysis (WGCNA) and machine learning. For WGCNA, consensus modules were detected and correlated with the single sample gene set enrichment analysis (ssGSEA) scores. Overlapping the differentially expressed genes (DEGs, Braak stages 0 vs. I-VI) with that in the interest module, metascape analysis, and Random Forest were conducted to explore the function of overlapping genes and obtain the most significant genes. We found that the three brain regions have high similarities in the gene expression pattern and that oxidative damage plays a vital role in NFT formation via machine learning. Through further filtering of genes from interested modules by Random Forest, we screened out key genes, such as LYN, LAPTM5, and IFI30. These key genes, including LYN, LAPTM5, and ARHGDIB, may play an important role in the development of AD through the inflammatory response pathway mediated by microglia.
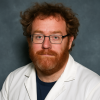
Dr. David Lowemann, M.Sc, Ph.D., is a co-founder of the Institute for the Future of Human Potential, where he leads the charge in pioneering Self-Enhancement Science for the Success of Society. With a keen interest in exploring the untapped potential of the human mind, Dr. Lowemann has dedicated his career to pushing the boundaries of human capabilities and understanding.
Armed with a Master of Science degree and a Ph.D. in his field, Dr. Lowemann has consistently been at the forefront of research and innovation, delving into ways to optimize human performance, cognition, and overall well-being. His work at the Institute revolves around a profound commitment to harnessing cutting-edge science and technology to help individuals lead more fulfilling and intelligent lives.
Dr. Lowemann’s influence extends to the educational platform BetterSmarter.me, where he shares his insights, findings, and personal development strategies with a broader audience. His ongoing mission is shaping the way we perceive and leverage the vast capacities of the human mind, offering invaluable contributions to society’s overall success and collective well-being.