Imagine if identifying schizophrenia was like solving a complex puzzle. Scientists have discovered that certain brainwave patterns, known as event-related potentials (ERPs), are linked to cognitive deficits in patients with schizophrenia. By applying machine learning techniques, like Multiple Kernel Learning (MKL) classifiers, researchers can find the optimal combination of these brainwave patterns to improve diagnosis accuracy. In a recent study, a cohort of individuals with schizophrenia and healthy controls were analyzed using three sets of ERP features. The Boruta feature selection method was used to identify the most influential variables, which turned out to be related to the timing and intensity of auditory responses. The results were impressive – an overall classification accuracy of 86% was achieved after applying the Boruta feature selection. This research not only reaffirms the potential of machine learning in mental health diagnosis but also highlights how sophisticated algorithms can significantly enhance our understanding of complex brain disorders. Curious to learn more? Check out the full article to delve into the fascinating world of machine learning and schizophrenia diagnosis!
AntecedentThe event-related potential (ERP) components P300 and mismatch negativity (MMN) have been linked to cognitive deficits in patients with schizophrenia. The diagnosis of schizophrenia could be improved by applying machine learning procedures to these objective neurophysiological biomarkers. Several studies have attempted to achieve this goal, but no study has examined Multiple Kernel Learning (MKL) classifiers. This algorithm finds optimally a combination of kernel functions, integrating them in a meaningful manner, and thus could improve diagnosis.ObjectiveThis study aimed to examine the efficacy of the MKL classifier and the Boruta feature selection method for schizophrenia patients (SZ) and healthy controls (HC) single-subject classification.MethodsA cohort of 54 SZ and 54 HC participants were studied. Three sets of features related to ERP signals were calculated as follows: peak related features, peak to peak related features, and signal related features. The Boruta algorithm was used to evaluate the impact of feature selection on classification performance. An MKL algorithm was applied to address schizophrenia detection.ResultsA classification accuracy of 83% using the whole dataset, and 86% after applying Boruta feature selection was obtained. The variables that contributed most to the classification were mainly related to the latency and amplitude of the auditory P300 paradigm.ConclusionThis study showed that MKL can be useful in distinguishing between schizophrenic patients and controls when using ERP measures. Moreover, the use of the Boruta algorithm provides an improvement in classification accuracy and computational cost.
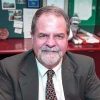
Dr. David Lowemann, M.Sc, Ph.D., is a co-founder of the Institute for the Future of Human Potential, where he leads the charge in pioneering Self-Enhancement Science for the Success of Society. With a keen interest in exploring the untapped potential of the human mind, Dr. Lowemann has dedicated his career to pushing the boundaries of human capabilities and understanding.
Armed with a Master of Science degree and a Ph.D. in his field, Dr. Lowemann has consistently been at the forefront of research and innovation, delving into ways to optimize human performance, cognition, and overall well-being. His work at the Institute revolves around a profound commitment to harnessing cutting-edge science and technology to help individuals lead more fulfilling and intelligent lives.
Dr. Lowemann’s influence extends to the educational platform BetterSmarter.me, where he shares his insights, findings, and personal development strategies with a broader audience. His ongoing mission is shaping the way we perceive and leverage the vast capacities of the human mind, offering invaluable contributions to society’s overall success and collective well-being.