In the quest to find new treatments for Alzheimer’s disease, scientists are searching for potential candidate compounds using a groundbreaking algorithm called forgeNet_SVM. By mining data from literature databases, researchers collect a mix of Alzheimer-related and unrelated compounds, which are then subjected to three different molecular descriptors to create feature sets. These feature sets are fused together into an all-encompassing feature set, which is fed into forgeNet_SVM. The forgeNet component analyzes the importance of each feature and selects the most crucial ones for further analysis. The selected features are then input into support vector machines (SVM) algorithm to identify unknown compounds from Traditional Chinese Medicine (TCM) prescriptions. The experiment demonstrates that the selected feature set outperforms both the all_feature set and three single-feature sets. This indicates that forgeNet_SVM is a more accurate classifier for identifying Alzheimer-related compounds. Overall, this research presents a promising approach to rapidly screening and identifying potential compounds for Alzheimer’s drug discovery. For a deeper dive into the study, be sure to check out the full article!
Rapid screening and identification of potential candidate compounds are very important to understand the mechanism of drugs for the treatment of Alzheimer’s disease (AD) and greatly promote the development of new drugs. In order to greatly improve the success rate of screening and reduce the cost and workload of research and development, this study proposes a novel Alzheimer-related compound identification algorithm namely forgeNet_SVM. First, Alzheimer related and unrelated compounds are collected using the data mining method from the literature databases. Three molecular descriptors (ECFP6, MACCS, and RDKit) are utilized to obtain the feature sets of compounds, which are fused into the all_feature set. The all_feature set is input to forgeNet_SVM, in which forgeNet is utilized to provide the importance of each feature and select the important features for feature extraction. The selected features are input to support vector machines (SVM) algorithm to identify the new compounds in Traditional Chinese Medicine (TCM) prescription. The experiment results show that the selected feature set performs better than the all_feature set and three single feature sets (ECFP6, MACCS, and RDKit). The performances of TPR, FPR, Precision, Specificity, F1, and AUC reveal that forgeNet_SVM could identify more accurately Alzheimer-related compounds than other classical classifiers.
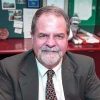
Dr. David Lowemann, M.Sc, Ph.D., is a co-founder of the Institute for the Future of Human Potential, where he leads the charge in pioneering Self-Enhancement Science for the Success of Society. With a keen interest in exploring the untapped potential of the human mind, Dr. Lowemann has dedicated his career to pushing the boundaries of human capabilities and understanding.
Armed with a Master of Science degree and a Ph.D. in his field, Dr. Lowemann has consistently been at the forefront of research and innovation, delving into ways to optimize human performance, cognition, and overall well-being. His work at the Institute revolves around a profound commitment to harnessing cutting-edge science and technology to help individuals lead more fulfilling and intelligent lives.
Dr. Lowemann’s influence extends to the educational platform BetterSmarter.me, where he shares his insights, findings, and personal development strategies with a broader audience. His ongoing mission is shaping the way we perceive and leverage the vast capacities of the human mind, offering invaluable contributions to society’s overall success and collective well-being.