Imagine you have a magical x-ray machine that can peer into someone’s brain and instantly identify signs of Parkinson’s disease. Well, that’s essentially what this new study has accomplished! Using cutting-edge deep learning algorithms, scientists have trained a convolutional neural network to analyze images generated by a specialized imaging technique called DaTscan. The network can accurately pinpoint the specific areas of the brain associated with Parkinson’s disease. By incorporating a soft-attention block into the architecture of DenseNet-121, the model achieves an impressive accuracy of 99.2% in distinguishing between PD and normal cases. The attention maps generated by the model highlight the putamen and caudate regions, which are crucial for Parkinson’s diagnosis. With such groundbreaking results, this research opens up new possibilities for early detection and tailored treatment plans for individuals with PD. To dive deeper into the details, check out the original research article!
ObjectiveDeep learning algorithms have long been involved in the diagnosis of severe neurological disorders that interfere with patients’ everyday tasks, such as Parkinson’s disease (PD). The most effective imaging modality for detecting the condition is DaTscan, a variety of single-photon emission computerized tomography (SPECT) imaging method. The goal is to create a convolutional neural network that can specifically identify the region of interest following feature extraction.MethodsThe study comprised a total of 1,390 DaTscan imaging groups with PD and normal classes. The architecture of DenseNet-121 is leveraged with a soft-attention block added before the final classification layer. For visually analyzing the region of interest (ROI) from the images after classification, Soft Attention Maps and feature map representation are used.OutcomesThe model obtains an overall accuracy of 99.2% and AUC-ROC score 99%. A sensitivity of 99.2%, specificity of 99.4% and f1-score of 99.1% is achieved that surpasses all prior research findings. Soft-attention map and feature map representation aid in highlighting the ROI, with a specific attention on the putamen and caudate regions.ConclusionWith the deep learning framework adopted, DaTscan images reveal the putamen and caudate areas of the brain, which aid in the distinguishing of normal and PD cohorts with high accuracy and sensitivity.
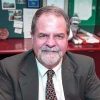
Dr. David Lowemann, M.Sc, Ph.D., is a co-founder of the Institute for the Future of Human Potential, where he leads the charge in pioneering Self-Enhancement Science for the Success of Society. With a keen interest in exploring the untapped potential of the human mind, Dr. Lowemann has dedicated his career to pushing the boundaries of human capabilities and understanding.
Armed with a Master of Science degree and a Ph.D. in his field, Dr. Lowemann has consistently been at the forefront of research and innovation, delving into ways to optimize human performance, cognition, and overall well-being. His work at the Institute revolves around a profound commitment to harnessing cutting-edge science and technology to help individuals lead more fulfilling and intelligent lives.
Dr. Lowemann’s influence extends to the educational platform BetterSmarter.me, where he shares his insights, findings, and personal development strategies with a broader audience. His ongoing mission is shaping the way we perceive and leverage the vast capacities of the human mind, offering invaluable contributions to society’s overall success and collective well-being.