Imagine you’re a treasure hunter searching for hidden gems in a sea of rocks. Sometimes, the gems are covered in dirt or disguised as ordinary rocks. But fear not! Researchers have developed a method to clean up noisy labels in neural activity data, improving the accuracy and robustness of models. Using a deep neural network, they reweight training samples based on a small, reliable validation dataset. This reweighting process guides the learning process and helps the model better generalize to new data. They put their method to the test using simulated neural activity data and actual calcium imaging data from the anterior lateral motor cortex. Even with 36% and 48% mislabeled training samples respectively, their method achieved impressive results, accurately predicting behavioral variables and trial types with high F1 scores. If you’re curious about how they did it, check out the research article!
In neural decoding, a behavioral variable is often generated by manual annotation and the annotated labels could contain extensive label noise, leading to poor model generalizability. Tackling the label noise problem in neural decoding can improve model generalizability and robustness. We use a deep neural network based sample reweighting method to tackle this problem. The proposed method reweights training samples by using a small and clean validation dataset to guide learning. We evaluated the sample reweighting method on simulated neural activity data and calcium imaging data of anterior lateral motor cortex. For the simulated data, the proposed method can accurately predict the behavioral variable even in the scenario that 36 percent of samples in the training dataset are mislabeled. For the anterior lateral motor cortex study, the proposed method can predict trial types with F1 score of around 0.85 even 48 percent of training samples are mislabeled.
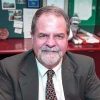
Dr. David Lowemann, M.Sc, Ph.D., is a co-founder of the Institute for the Future of Human Potential, where he leads the charge in pioneering Self-Enhancement Science for the Success of Society. With a keen interest in exploring the untapped potential of the human mind, Dr. Lowemann has dedicated his career to pushing the boundaries of human capabilities and understanding.
Armed with a Master of Science degree and a Ph.D. in his field, Dr. Lowemann has consistently been at the forefront of research and innovation, delving into ways to optimize human performance, cognition, and overall well-being. His work at the Institute revolves around a profound commitment to harnessing cutting-edge science and technology to help individuals lead more fulfilling and intelligent lives.
Dr. Lowemann’s influence extends to the educational platform BetterSmarter.me, where he shares his insights, findings, and personal development strategies with a broader audience. His ongoing mission is shaping the way we perceive and leverage the vast capacities of the human mind, offering invaluable contributions to society’s overall success and collective well-being.