Detecting early changes in the brain morphology and diagnosing Alzheimer’s disease (AD) are crucial, and magnetic resonance imaging (MRI) can assist in this process. Researchers have developed two enhanced ResNet algorithms that incorporate the Contextual Transformer (CoT) module, group convolution, and Channel Shuffle mechanism into the traditional ResNet architecture. The CoT module replaces the 3×3 convolution in the residual block to enhance feature extraction, while the Channel Shuffle mechanism rearranges the feature maps to improve communication between different groups. The algorithms were tested on MRI data from 503 subjects including healthy controls, mild cognitive impairment (MCI) patients, and AD patients. The results showed that the refined ResNet-18-based algorithm improved accuracy by percentages ranging from 0.33% to 2.06% in various medical image classification tasks, compared to the traditional ResNet-18 algorithm. Similarly, the enhanced ResNet-50-based algorithm achieved accuracy improvements of 1.02% to 3.30%. These findings highlight the effectiveness of incorporating the CoT module and Channel Shuffle mechanism in improving AD classification.
Detection of early morphological changes in the brain and early diagnosis are important for Alzheimer’s disease (AD), and high-resolution magnetic resonance imaging (MRI) can be used to help diagnose and predict the disease. In this paper, we proposed two improved ResNet algorithms that introduced the Contextual Transformer (CoT) module, group convolution, and Channel Shuffle mechanism into the traditional ResNet residual blocks. The CoT module is used to replace the 3 × 3 convolution in the residual block to enhance the feature extraction capability of the residual block, while the Channel Shuffle mechanism is used to reorganize the feature maps of different groups in the input layer to improve the communication between the feature maps from different groups. Images of 503 subjects, including 116 healthy controls (HC), 187 subjects with mild cognitive impairment (MCI), and 200 subjects with AD, were selected and collated from the ADNI database, and then, the data were pre-processed and sliced. After that, 10,060 slices were obtained and the three groups of AD, MCI and HC were classified using the improved algorithms. The experiments showed that the refined ResNet-18-based algorithm improved the top-1 accuracy by 2.06%, 0.33%, 1.82%, and 1.52% over the traditional ResNet-18 algorithm for four medical image classification tasks, namely AD: MCI, AD: HC, MCI: HC, and AD: MCI: HC, respectively. The enhanced ResNet-50-based algorithm improved the top-1 accuracy by 1.02%, 2.92%, 3.30%, and 1.31%, respectively, over the traditional ResNet-50 algorithm in four medical image classification tasks, demonstrating the effectiveness of the CoT module replacement and the inclusion of the channel shuffling mechanism, as well as the competitiveness of the improved algorithms.
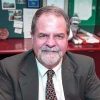
Dr. David Lowemann, M.Sc, Ph.D., is a co-founder of the Institute for the Future of Human Potential, where he leads the charge in pioneering Self-Enhancement Science for the Success of Society. With a keen interest in exploring the untapped potential of the human mind, Dr. Lowemann has dedicated his career to pushing the boundaries of human capabilities and understanding.
Armed with a Master of Science degree and a Ph.D. in his field, Dr. Lowemann has consistently been at the forefront of research and innovation, delving into ways to optimize human performance, cognition, and overall well-being. His work at the Institute revolves around a profound commitment to harnessing cutting-edge science and technology to help individuals lead more fulfilling and intelligent lives.
Dr. Lowemann’s influence extends to the educational platform BetterSmarter.me, where he shares his insights, findings, and personal development strategies with a broader audience. His ongoing mission is shaping the way we perceive and leverage the vast capacities of the human mind, offering invaluable contributions to society’s overall success and collective well-being.