Filtering out facial muscle noise from electroencephalographic (EEG) signals is essential for accurate brain computer interfaces and brain functionality measurement. While most studies focus on multi-channel approaches, this research introduces a single-channel method for attenuating facial electromyographic (EMG) noise from contaminated EEG. The method incorporates multiple decomposition and adaptive filtering techniques to generate reference signals and filter out the EMG interference. Different decomposition methods such as CiSSA, EMD, EEMD, EMD-PCA, SSA, and Wavelet were evaluated, along with adaptive filtering methods like RLS, Wiener, LMS, and NLMS. The study used features in the time and frequency domain to evaluate the performance of the single-channel method and characterize contamination from specific facial muscles. The Masseter muscle showed the highest level of contamination. The results indicate that the SSA method generated more suitable reference signals, while the RLS and NLMS methods were effective when the reference signal was derived from the EEG. Conversely, the LMS and RLS methods worked well when the reference signal was EMG-based. This research has practical implications for reducing EEG contamination caused by facial muscle activation in various types of studies. The developed code is available to reproduce and improve the results.
Eliminating facial electromyographic (EMG) signal from the electroencephalogram (EEG) is crucial for the accuracy of applications such as brain computer interfaces (BCIs) and brain functionality measurement. Facial electromyography typically corrupts the electroencephalogram. Although it is possible to find in the literature a number of multi-channel approaches for filtering corrupted EEG, studies employing single-channel approaches are scarce. In this context, this study proposed a single-channel method for attenuating facial EMG noise from contaminated EEG. The architecture of the method allows for the evaluation and incorporation of multiple decomposition and adaptive filtering techniques. The decomposition method was responsible for generating EEG or EMG reference signals for the adaptive filtering stage. In this study, the decomposition techniques CiSSA, EMD, EEMD, EMD-PCA, SSA, and Wavelet were evaluated. The adaptive filtering methods RLS, Wiener, LMS, and NLMS were investigated. A time and frequency domain set of features were estimated from experimental signals to evaluate the performance of the single channel method. This set of characteristics permitted the characterization of the contamination of distinct facial muscles, namely Masseter, Frontalis, Zygomatic, Orbicularis Oris, and Orbicularis Oculi. Data were collected from ten healthy subjects executing an experimental protocol that introduced the necessary variability to evaluate the filtering performance. The largest level of contamination was produced by the Masseter muscle, as determined by statistical analysis of the set of features and visualization of topological maps. Regarding the decomposition method, the SSA method allowed for the generation of more suitable reference signals, whereas the RLS and NLMS methods were more suitable when the reference signal was derived from the EEG. In addition, the LMS and RLS methods were more appropriate when the reference signal was the EMG. This study has a number of practical implications, including the use of filtering techniques to reduce EEG contamination caused by the activation of facial muscles required by distinct types of studies. All the developed code, including examples, is available to facilitate a more accurate reproduction and improvement of the results of this study.
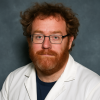
Dr. David Lowemann, M.Sc, Ph.D., is a co-founder of the Institute for the Future of Human Potential, where he leads the charge in pioneering Self-Enhancement Science for the Success of Society. With a keen interest in exploring the untapped potential of the human mind, Dr. Lowemann has dedicated his career to pushing the boundaries of human capabilities and understanding.
Armed with a Master of Science degree and a Ph.D. in his field, Dr. Lowemann has consistently been at the forefront of research and innovation, delving into ways to optimize human performance, cognition, and overall well-being. His work at the Institute revolves around a profound commitment to harnessing cutting-edge science and technology to help individuals lead more fulfilling and intelligent lives.
Dr. Lowemann’s influence extends to the educational platform BetterSmarter.me, where he shares his insights, findings, and personal development strategies with a broader audience. His ongoing mission is shaping the way we perceive and leverage the vast capacities of the human mind, offering invaluable contributions to society’s overall success and collective well-being.