Just like unraveling the intricate web of brain networks during natural speech comprehension, scientists are delving into the functional mechanism of language processing. By using a technique called temporal response functions (TRFs) on electroencephalograph (EEG) data, researchers can estimate the neural sources and investigate the brain networks involved in understanding speech. This method involves reducing EEG noise through a functional hyper-alignment method and reconstructing neural sources from the EEG signals. The detected brain networks for normal speech comprehension were found to be distinctly different from those for non-semantically driven audio processing. These findings suggest that the proposed TRFs capture the cognitive processing of spoken language. To gain a comprehensive understanding, researchers applied a multi-scale community detection method to identify brain network communities at different scales. It’s exciting to see how exploring these brain networks brings us closer to uncovering the secrets of speech comprehension.
In recent years, electroencephalograph (EEG) studies on speech comprehension have been extended from a controlled paradigm to a natural paradigm. Under the hypothesis that the brain can be approximated as a linear time-invariant system, the neural response to natural speech has been investigated extensively using temporal response functions (TRFs). However, most studies have modeled TRFs in the electrode space, which is a mixture of brain sources and thus cannot fully reveal the functional mechanism underlying speech comprehension. In this paper, we propose methods for investigating the brain networks of natural speech comprehension using TRFs on the basis of EEG source reconstruction. We first propose a functional hyper-alignment method with an additive average method to reduce EEG noise. Then, we reconstruct neural sources within the brain based on the EEG signals to estimate TRFs from speech stimuli to source areas, and then investigate the brain networks in the neural source space on the basis of the community detection method. To evaluate TRF-based brain networks, EEG data were recorded in story listening tasks with normal speech and time-reversed speech. To obtain reliable structures of brain networks, we detected TRF-based communities from multiple scales. As a result, the proposed functional hyper-alignment method could effectively reduce the noise caused by individual settings in an EEG experiment and thus improve the accuracy of source reconstruction. The detected brain networks for normal speech comprehension were clearly distinctive from those for non-semantically driven (time-reversed speech) audio processing. Our result indicates that the proposed source TRFs can reflect the cognitive processing of spoken language and that the multi-scale community detection method is powerful for investigating brain networks.
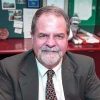
Dr. David Lowemann, M.Sc, Ph.D., is a co-founder of the Institute for the Future of Human Potential, where he leads the charge in pioneering Self-Enhancement Science for the Success of Society. With a keen interest in exploring the untapped potential of the human mind, Dr. Lowemann has dedicated his career to pushing the boundaries of human capabilities and understanding.
Armed with a Master of Science degree and a Ph.D. in his field, Dr. Lowemann has consistently been at the forefront of research and innovation, delving into ways to optimize human performance, cognition, and overall well-being. His work at the Institute revolves around a profound commitment to harnessing cutting-edge science and technology to help individuals lead more fulfilling and intelligent lives.
Dr. Lowemann’s influence extends to the educational platform BetterSmarter.me, where he shares his insights, findings, and personal development strategies with a broader audience. His ongoing mission is shaping the way we perceive and leverage the vast capacities of the human mind, offering invaluable contributions to society’s overall success and collective well-being.