Just like finding a hidden treasure map can lead to great rewards, early detection of Alzheimer’s disease can help control its progression. But current detection techniques are either expensive, hard to access, or invasive. That’s why scientists have been exploring a new approach using resting state electroencephalography (EEG), which is safe and easy to use. By combining it with machine learning algorithms, they were able to create an automated system for detecting Alzheimer’s disease. This system analyzed the brain activity of 26 participants from different groups and used advanced algorithms to identify specific features related to the disease. The exciting results showed that their automated approach produced similar outcomes as manual procedures, but without the need for extra personnel or complex EEG systems. This means that by using commercial EEG systems and automated analysis, doctors may be able to detect Alzheimer’s disease more efficiently and start medical treatment sooner. The potential impact of this research is enormous in terms of reducing costs and accelerating the detection and treatment of Alzheimer’s disease.
Early detection is crucial to control the progression of Alzheimer’s disease and to postpone intellectual decline. Most current detection techniques are costly, inaccessible, or invasive. Furthermore, they require laborious analysis, what delays the start of medical treatment. To overcome this, researchers have recently investigated AD detection based on electroencephalography, a non-invasive neurophysiology technique, and machine learning algorithms. However, these approaches typically rely on manual procedures such as visual inspection, that requires additional personnel for the analysis, or on cumbersome EEG acquisition systems. In this paper, we performed a preliminary evaluation of a fully-automated approach for AD detection based on a commercial EEG acquisition system and an automated classification pipeline. For this purpose, we recorded the resting state brain activity of 26 participants from three groups: mild AD, mild cognitive impairment (MCI-non-AD), and healthy controls. First, we applied automated data-driven algorithms to reject EEG artifacts. Then, we obtained spectral, complexity, and entropy features from the preprocessed EEG segments. Finally, we assessed two binary classification problems: mild AD vs. controls, and MCI-non-AD vs. controls, through leave-one-subject-out cross-validation. The preliminary results that we obtained are comparable to the best reported in literature, what suggests that AD detection could be automatically detected through automated processing and commercial EEG systems. This is promising, since it may potentially contribute to reducing costs related to AD screening, and to shortening detection times, what may help to advance medical treatment.
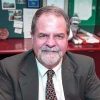
Dr. David Lowemann, M.Sc, Ph.D., is a co-founder of the Institute for the Future of Human Potential, where he leads the charge in pioneering Self-Enhancement Science for the Success of Society. With a keen interest in exploring the untapped potential of the human mind, Dr. Lowemann has dedicated his career to pushing the boundaries of human capabilities and understanding.
Armed with a Master of Science degree and a Ph.D. in his field, Dr. Lowemann has consistently been at the forefront of research and innovation, delving into ways to optimize human performance, cognition, and overall well-being. His work at the Institute revolves around a profound commitment to harnessing cutting-edge science and technology to help individuals lead more fulfilling and intelligent lives.
Dr. Lowemann’s influence extends to the educational platform BetterSmarter.me, where he shares his insights, findings, and personal development strategies with a broader audience. His ongoing mission is shaping the way we perceive and leverage the vast capacities of the human mind, offering invaluable contributions to society’s overall success and collective well-being.