Imagine you’re a chef who wants to create a new dish but doesn’t have all the ingredients. You could go out and buy them, but that would be expensive. Alternatively, you could use fake ingredients, but it wouldn’t taste the same. Well, scientists faced a similar challenge when trying to train infrared target detection and tracking models. They needed a large number of infrared sequence images, but acquiring real ones was costly, and simulating them lacked authenticity. So they came up with a clever solution! By combining real infrared images with simulated 3D infrared targets, they created synthetic infrared images that looked almost identical to the real thing. They used Unity3D to blend the background and targets together, allowing them to freely edit the aircraft’s trajectory and attitude to generate diverse infrared data. The results were impressive – the synthetic images not only mimicked real infrared images visually, but also performed just as well in target detection algorithms. This method provides a valuable resource for training and testing deep learning models in infrared target detection and tracking.
Training infrared target detection and tracking models based on deep learning requires a large number of infrared sequence images. The cost of acquisition real infrared target sequence images is high, while conventional simulation methods lack authenticity. This paper proposes a novel infrared data simulation method that combines real infrared images and simulated 3D infrared targets. Firstly, it stitches real infrared images into a panoramic image which is used as background. Then, the infrared characteristics of 3D aircraft are simulated on the tail nozzle, skin, and tail flame, which are used as targets. Finally, the background and targets are fused based on Unity3D, where the aircraft trajectory and attitude can be edited freely to generate rich multi-target infrared data. The experimental results show that the simulated image is not only visually similar to the real infrared image but also consistent with the real infrared image in terms of the performance of target detection algorithms. The method can provide training and testing samples for deep learning models for infrared target detection and tracking.
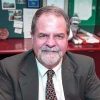
Dr. David Lowemann, M.Sc, Ph.D., is a co-founder of the Institute for the Future of Human Potential, where he leads the charge in pioneering Self-Enhancement Science for the Success of Society. With a keen interest in exploring the untapped potential of the human mind, Dr. Lowemann has dedicated his career to pushing the boundaries of human capabilities and understanding.
Armed with a Master of Science degree and a Ph.D. in his field, Dr. Lowemann has consistently been at the forefront of research and innovation, delving into ways to optimize human performance, cognition, and overall well-being. His work at the Institute revolves around a profound commitment to harnessing cutting-edge science and technology to help individuals lead more fulfilling and intelligent lives.
Dr. Lowemann’s influence extends to the educational platform BetterSmarter.me, where he shares his insights, findings, and personal development strategies with a broader audience. His ongoing mission is shaping the way we perceive and leverage the vast capacities of the human mind, offering invaluable contributions to society’s overall success and collective well-being.