Imagine your brain is a bustling city with different neighborhoods communicating through intricate networks of roads. In the case of mild cognitive impairment (MCI), Alzheimer’s disease poses a threat to these pathways. To improve the classification of MCI using machine learning, scientists focus on the hippocampus, an essential hub in the brain’s white matter network. By examining structural and functional connections in the white matter surrounding the hippocampus, researchers can extract key features for diagnosis. Using MRI imaging techniques, they identified regions that show strong connectivity to the hippocampus, creating a hippocampus-related white matter network. By comparing the classification performances of different networks, they discovered that the hippocampus-related network provided the best accuracy (89.4%) and area under the curve (AUC) of 0.954. Notably, they observed that both the hippocampus and thalamus play crucial roles in MCI’s pathological mechanism. These findings contribute to early detection and diagnosis of Alzheimer’s disease before it fully develops. Curious to explore this fascinating research? Dive into the full article for more!
BackgroundDetection of mild cognitive impairment (MCI) is essential to screen high risk of Alzheimer’s disease (AD). However, subtle changes during MCI make it challenging to classify in machine learning. The previous pathological analysis pointed out that the hippocampus is the critical hub for the white matter (WM) network of MCI. Damage to the white matter pathways around the hippocampus is the main cause of memory decline in MCI. Therefore, it is vital to biologically extract features from the WM network driven by hippocampus-related regions to improve classification performance.MethodsOur study proposes a method for feature extraction of the whole-brain WM network. First, 42 MCI and 54 normal control (NC) subjects were recruited using diffusion tensor imaging (DTI), resting-state functional magnetic resonance imaging (rs-fMRI), and T1-weighted (T1w) imaging. Second, mean diffusivity (MD) and fractional anisotropy (FA) were calculated from DTI, and the whole-brain WM networks were obtained. Third, regions of interest (ROIs) with significant functional connectivity to the hippocampus were selected for feature extraction, and the hippocampus (HIP)-related WM networks were obtained. Furthermore, the rank sum test with Bonferroni correction was used to retain significantly different connectivity between MCI and NC, and significant HIP-related WM networks were obtained. Finally, the classification performances of these three WM networks were compared to select the optimal feature and classifier.Results(1) For the features, the whole-brain WM network, HIP-related WM network, and significant HIP-related WM network are significantly improved in turn. Also, the accuracy of MD networks as features is better than FA. (2) For the classification algorithm, the support vector machine (SVM) classifier with radial basis function, taking the significant HIP-related WM network in MD as a feature, has the optimal classification performance (accuracy = 89.4%, AUC = 0.954). (3) For the pathologic mechanism, the hippocampus and thalamus are crucial hubs of the WM network for MCI.ConclusionFeature extraction from the WM network driven by hippocampus-related regions provides an effective method for the early diagnosis of AD.
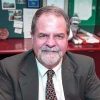
Dr. David Lowemann, M.Sc, Ph.D., is a co-founder of the Institute for the Future of Human Potential, where he leads the charge in pioneering Self-Enhancement Science for the Success of Society. With a keen interest in exploring the untapped potential of the human mind, Dr. Lowemann has dedicated his career to pushing the boundaries of human capabilities and understanding.
Armed with a Master of Science degree and a Ph.D. in his field, Dr. Lowemann has consistently been at the forefront of research and innovation, delving into ways to optimize human performance, cognition, and overall well-being. His work at the Institute revolves around a profound commitment to harnessing cutting-edge science and technology to help individuals lead more fulfilling and intelligent lives.
Dr. Lowemann’s influence extends to the educational platform BetterSmarter.me, where he shares his insights, findings, and personal development strategies with a broader audience. His ongoing mission is shaping the way we perceive and leverage the vast capacities of the human mind, offering invaluable contributions to society’s overall success and collective well-being.