Imagine trying to navigate through a dense forest without a map or compass. That’s similar to the challenges faced by scientists trying to diagnose Alzheimer’s disease (AD). AD is a progressive brain disorder that causes memory loss and cognitive decline. The current diagnostic methods are limited, so researchers are constantly searching for better approaches. In this study, scientists collected gene expression data from the Gene Expression Omnibus database and used advanced techniques like random forest (RF) and artificial neural network (ANN) to develop a combined diagnostic model for AD. They identified six key genes that play a crucial role in differentiating AD from normal samples. With an impressive accuracy of 91.4% and an area under the curve (AUC) of 0.953, the diagnostic model showed great promise! But the journey didn’t end there. The model was tested on two validation datasets, GSE109887 and GSE132903, and demonstrated good performance with AUC values of 0.854 and 0.810 respectively. By unraveling the genetic biomarkers associated with AD, this study sheds light on the complex nature of the disease and offers new avenues for early detection and intervention. If you’re curious about the exciting research, check out the full article!
Alzheimer’s disease (AD) is a neurodegenerative condition that causes cognitive decline over time. Because existing diagnostic approaches for AD are limited, improving upon previously established diagnostic models based on genetic biomarkers is necessary. Firstly, four AD gene expression datasets were collected from the Gene Expression Omnibus (GEO) database. Two datasets were used to establish diagnostic models, and the other two datasets were used to verify the model effect. We merged GSE5281 with GSE44771 as the training dataset and found 120 DEGs. Then, we used random forest (RF) to screen 6 key genes (KLF15, MAFF, ITPKB, SST, DDIT4, and NRXN3) as being critical for separating AD and normal samples. The weights of these key genes were measured, and a diagnostic model was created using an artificial neural network (ANN). The area under the curve (AUC) of the model is 0.953, while the accuracy is 0.914. In the final step, two validation datasets were utilized to assess AUC performance. In GSE109887, our model had an AUC of 0.854, and in GSE132903, it had an AUC of 0.810. To summarize, we successfully identified key gene biomarkers and developed a new AD diagnostic model.
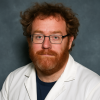
Dr. David Lowemann, M.Sc, Ph.D., is a co-founder of the Institute for the Future of Human Potential, where he leads the charge in pioneering Self-Enhancement Science for the Success of Society. With a keen interest in exploring the untapped potential of the human mind, Dr. Lowemann has dedicated his career to pushing the boundaries of human capabilities and understanding.
Armed with a Master of Science degree and a Ph.D. in his field, Dr. Lowemann has consistently been at the forefront of research and innovation, delving into ways to optimize human performance, cognition, and overall well-being. His work at the Institute revolves around a profound commitment to harnessing cutting-edge science and technology to help individuals lead more fulfilling and intelligent lives.
Dr. Lowemann’s influence extends to the educational platform BetterSmarter.me, where he shares his insights, findings, and personal development strategies with a broader audience. His ongoing mission is shaping the way we perceive and leverage the vast capacities of the human mind, offering invaluable contributions to society’s overall success and collective well-being.