Alzheimer’s Disease is like an intricate puzzle that scientists are determined to solve. In order to understand and effectively treat this progressive neurodegenerative disease, researchers have developed a method called MPC-STANet. This method uses a combination of techniques, including Multi-Phantom Convolution (MPC) and Space Conversion Attention Mechanism (MPC-STANet), to recognize different stages of Alzheimer’s disease. Just like putting together puzzle pieces, the researchers employ class-balancing operations and data expansion techniques to create a balanced dataset for training. They then use ResNet50 as the backbone network and introduce Multi-Phantom Residual Blocks (MPRB) to better identify the scattered and tiny disease features. Additionally, the Space Conversion Attention Mechanism (SCAM) helps detect subtle structural changes within MRI images of AD patients. The experimental results showed impressive accuracy rates for the proposed method. With an average recognition accuracy of 96.25% and a number of parameters only slightly more than ResNet50, MPC-STANet shows great promise in the ongoing efforts to understand and combat Alzheimer’s disease. For more details, check out the full article!
Alzheimer’s disease (AD) is a progressive neurodegenerative disease with insidious and irreversible onset. The recognition of the disease stage of AD and the administration of effective interventional treatment are important to slow down and control the progression of the disease. However, due to the unbalanced distribution of the acquired data volume, the problem that the features change inconspicuously in different disease stages of AD, and the scattered and narrow areas of the feature areas (hippocampal region, medial temporal lobe, etc.), the effective recognition of AD remains a critical unmet need. Therefore, we first employ class-balancing operation using data expansion and Synthetic Minority Oversampling Technique (SMOTE) to avoid the AD MRI dataset being affected by classification imbalance in the training. Subsequently, a recognition network based on Multi-Phantom Convolution (MPC) and Space Conversion Attention Mechanism (MPC-STANet) with ResNet50 as the backbone network is proposed for the recognition of the disease stages of AD. In this study, we propose a Multi-Phantom Convolution in the way of convolution according to the channel direction and integrate it with the average pooling layer into two basic blocks of ResNet50: Conv Block and Identity Block to propose the Multi-Phantom Residual Block (MPRB) including Multi-Conv Block and Multi-Identity Block to better recognize the scattered and tiny disease features of Alzheimer’s disease. Meanwhile, the weight coefficients are extracted from both vertical and horizontal directions using the Space Conversion Attention Mechanism (SCAM) to better recognize subtle structural changes in the AD MRI images. The experimental results show that our proposed method achieves an average recognition accuracy of 96.25%, F1 score of 95%, and mAP of 93%, and the number of parameters is only 1.69 M more than ResNet50.
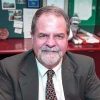
Dr. David Lowemann, M.Sc, Ph.D., is a co-founder of the Institute for the Future of Human Potential, where he leads the charge in pioneering Self-Enhancement Science for the Success of Society. With a keen interest in exploring the untapped potential of the human mind, Dr. Lowemann has dedicated his career to pushing the boundaries of human capabilities and understanding.
Armed with a Master of Science degree and a Ph.D. in his field, Dr. Lowemann has consistently been at the forefront of research and innovation, delving into ways to optimize human performance, cognition, and overall well-being. His work at the Institute revolves around a profound commitment to harnessing cutting-edge science and technology to help individuals lead more fulfilling and intelligent lives.
Dr. Lowemann’s influence extends to the educational platform BetterSmarter.me, where he shares his insights, findings, and personal development strategies with a broader audience. His ongoing mission is shaping the way we perceive and leverage the vast capacities of the human mind, offering invaluable contributions to society’s overall success and collective well-being.