In the world of neuroscience and neurology, analyzing neuroimages has become a powerful tool. However, a major challenge arises when dealing with different protocols and vendors, resulting in incompatible data known as ‘center effects.’ To address this issue, researchers developed a linear mixed effect model to harmonize cortical features obtained from multicenter MRI data. By correcting for biological variables and considering center information as a random effect, they successfully corrected the center effects. The results showed that the model-based w-score outperformed raw cortical thickness in predicting dataset origin and classifying normal and patient groups. Additionally, comparisons with existing methods and disease atrophy patterns such as Alzheimer’s and Parkinson’s revealed the effectiveness of the model in preserving disease-related effects. Overall, this study presents a promising approach to harmonizing multicenter cortical thickness data, providing researchers with standardized language for exploring the mysteries of the brain.
ObjectiveAnalyzing neuroimages being useful method in the field of neuroscience and neurology and solving the incompatibilities across protocols and vendors have become a major problem. We referred to this incompatibility as “center effects,” and in this study, we attempted to correct such center effects of cortical feature obtained from multicenter magnetic resonance images (MRIs).MethodsFor MRI of a total of 4,321 multicenter subjects, the harmonized w-score was calculated by correcting biological covariates such as age, sex, years of education, and intercranial volume (ICV) as fixed effects and center information as a random effect. Afterward, we performed classification tasks using principal component analysis (PCA) and linear discriminant analysis (LDA) to check whether the center effect was successfully corrected from the harmonized w-score.ResultsFirst, an experiment was conducted to predict the dataset origin of a random subject sampled from two different datasets, and it was confirmed that the prediction accuracy of linear mixed effect (LME) model-based w-score was significantly closer to the baseline than that of raw cortical thickness. As a second experiment, we classified the data of the normal and patient groups of each dataset, and LME model-based w-score, which is biological-feature-corrected values, showed higher classification accuracy than the raw cortical thickness data. Afterward, to verify the compatibility of the dataset used for LME model training and the dataset that is not, intraobject comparison and w-score RMSE calculation process were performed.ConclusionThrough comparison between the LME model-based w-score and existing methods and several classification tasks, we showed that the LME model-based w-score sufficiently corrects the center effects while preserving the disease effects from the dataset. We also showed that the preserved disease effects have a match with well-known disease atrophy patterns such as Alzheimer’s disease or Parkinson’s disease. Finally, through intrasubject comparison, we found that the difference between centers decreases in the LME model-based w-score compared with the raw cortical thickness and thus showed that our model well-harmonizes the data that are not used for the model training.
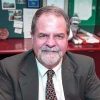
Dr. David Lowemann, M.Sc, Ph.D., is a co-founder of the Institute for the Future of Human Potential, where he leads the charge in pioneering Self-Enhancement Science for the Success of Society. With a keen interest in exploring the untapped potential of the human mind, Dr. Lowemann has dedicated his career to pushing the boundaries of human capabilities and understanding.
Armed with a Master of Science degree and a Ph.D. in his field, Dr. Lowemann has consistently been at the forefront of research and innovation, delving into ways to optimize human performance, cognition, and overall well-being. His work at the Institute revolves around a profound commitment to harnessing cutting-edge science and technology to help individuals lead more fulfilling and intelligent lives.
Dr. Lowemann’s influence extends to the educational platform BetterSmarter.me, where he shares his insights, findings, and personal development strategies with a broader audience. His ongoing mission is shaping the way we perceive and leverage the vast capacities of the human mind, offering invaluable contributions to society’s overall success and collective well-being.