Imagine a group of dancers trying to synchronize their moves on a crowded dance floor. They follow a specific rhythm and rely on visual cues to stay in sync. Now, picture this scenario playing out inside our brains, where groups of neurons perform a complex dance of communication. In a recent study, scientists explored the intricate dynamics between two well-known models – the Kuramoto-Sakaguchi model and Hebb dynamics – to understand how synchronized states emerge. What they discovered is fascinating: under certain conditions, a mesmerizing phenomenon called the ‘chimera pattern’ can occur within the Hebbian synaptic strengths. This pattern arises when two different regions of oscillators rotate at different speeds – one moving slower, while the other spins faster. By adjusting the parameters that govern frustration and introducing a pacemaker oscillator, researchers created non-stationary behavior reminiscent of hysteresis and observed a switch-like phenomenon similar to how we learn and remember things. The implications of this research could help us unravel more secrets about the brain’s incredible capacity for information processing and storage! To delve into the mesmerizing world of chimera patterns and Hebb synapses, check out the full article.
The union of the Kuramoto–Sakaguchi model and the Hebb dynamics reproduces the Lisman switch through a bistability in synchronized states. Here, we show that, within certain ranges of the frustration parameter, the chimera pattern can emerge, causing a different, time-evolving, distribution in the Hebbian synaptic strengths. We study the stability range of the chimera as a function of the frustration (phase-lag) parameter. Depending on the range of the frustration, two different types of chimeras can appear spontaneously, i.e., from randomized initial conditions. In the first type, the oscillators in the coherent region rotate, on average, slower than those in the incoherent region; while in the second type, the average rotational frequencies of the two regions are reversed, i.e., the coherent region runs, on average, faster than the incoherent region. We also show that non-stationary behavior at finite N can be controlled by adjusting the natural frequency of a single pacemaker oscillator. By slowly cycling the frequency of the pacemaker, we observe hysteresis in the system. Finally, we discuss how we can have a model for learning and memory.
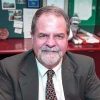
Dr. David Lowemann, M.Sc, Ph.D., is a co-founder of the Institute for the Future of Human Potential, where he leads the charge in pioneering Self-Enhancement Science for the Success of Society. With a keen interest in exploring the untapped potential of the human mind, Dr. Lowemann has dedicated his career to pushing the boundaries of human capabilities and understanding.
Armed with a Master of Science degree and a Ph.D. in his field, Dr. Lowemann has consistently been at the forefront of research and innovation, delving into ways to optimize human performance, cognition, and overall well-being. His work at the Institute revolves around a profound commitment to harnessing cutting-edge science and technology to help individuals lead more fulfilling and intelligent lives.
Dr. Lowemann’s influence extends to the educational platform BetterSmarter.me, where he shares his insights, findings, and personal development strategies with a broader audience. His ongoing mission is shaping the way we perceive and leverage the vast capacities of the human mind, offering invaluable contributions to society’s overall success and collective well-being.