Think of your brain as a grand treasure map, hiding the secrets to Alzheimer’s disease. Scientists have long been on the hunt to uncover the regions within our brains that are associated with this devastating condition. In a groundbreaking study, researchers utilized an artificial intelligence model to explore the uncharted territory of whole brain segmented regions. By analyzing radiomic features from these regions, they were able to pinpoint the specific subregions most closely linked to Alzheimer’s disease. These included the hippocampus, inferior parietal lobe, precuneus, and lateral occipital gyrus – each holding valuable clues. Shape, gray level size zone matrix (GLSZM), and gray level dependence matrix (GLDM) were just a few of the important features uncovered. Armed with this knowledge, the scientists built an integrated model using the powerful Logistic regression algorithm, achieving an incredible accuracy rate of 96.2%! This remarkable breakthrough paves the way for potential biomarkers that could revolutionize early detection and prediction of Alzheimer’s disease.
Alzheimer’s disease (AD) is the most common form of dementia, causing progressive cognitive decline. Radiomic features obtained from structural magnetic resonance imaging (sMRI) have shown a great potential in predicting this disease. However, radiomic features based on the whole brain segmented regions have not been explored yet. In our study, we collected sMRI data that include 80 patients with AD and 80 healthy controls (HCs). For each patient, the T1 weighted image (T1WI) images were segmented into 106 subregions, and radiomic features were extracted from each subregion. Then, we analyzed the radiomic features of specific brain subregions that were most related to AD. Based on the selective radiomic features from specific brain subregions, we built an integrated model using the best machine learning algorithms, and the diagnostic accuracy was evaluated. The subregions most relevant to AD included the hippocampus, the inferior parietal lobe, the precuneus, and the lateral occipital gyrus. These subregions exhibited several important radiomic features that include shape, gray level size zone matrix (GLSZM), and gray level dependence matrix (GLDM), among others. Based on the comparison among different algorithms, we constructed the best model using the Logistic regression (LR) algorithm, which reached an accuracy of 0.962. Conclusively, we constructed an excellent model based on radiomic features from several specific AD-related subregions, which could give a potential biomarker for predicting AD.
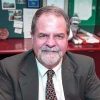
Dr. David Lowemann, M.Sc, Ph.D., is a co-founder of the Institute for the Future of Human Potential, where he leads the charge in pioneering Self-Enhancement Science for the Success of Society. With a keen interest in exploring the untapped potential of the human mind, Dr. Lowemann has dedicated his career to pushing the boundaries of human capabilities and understanding.
Armed with a Master of Science degree and a Ph.D. in his field, Dr. Lowemann has consistently been at the forefront of research and innovation, delving into ways to optimize human performance, cognition, and overall well-being. His work at the Institute revolves around a profound commitment to harnessing cutting-edge science and technology to help individuals lead more fulfilling and intelligent lives.
Dr. Lowemann’s influence extends to the educational platform BetterSmarter.me, where he shares his insights, findings, and personal development strategies with a broader audience. His ongoing mission is shaping the way we perceive and leverage the vast capacities of the human mind, offering invaluable contributions to society’s overall success and collective well-being.