Developing biophysical neuronal models is like constructing a detailed blueprint of the brain’s neural networks. Researchers face the challenge of accurately representing ion channel dynamics in these models. A solution comes in the form of NeuroGPU-EA, an innovative algorithm that harnesses the power of both CPUs and GPUs. It simulates and evaluates neuron membrane potentials with multiple stimuli, helping to refine the models efficiently. In fact, NeuroGPU-EA outshines the traditional CPU-based evolutionary algorithm by a whopping tenfold! Scaling benchmarks show a logarithmic cost as stimuli increase in complexity, making this cutting-edge method even more impressive. The researchers delve into the performance bottlenecks encountered and suggest strategies to overcome them. Additionally, they highlight how this approach could revolutionize simulations and evaluations of electrophysiological waveforms. If you’re curious about the intricacies of biophysical neural modeling and want to explore this groundbreaking research further, don’t miss out on the full article!
Single neuron models are fundamental for computational modeling of the brain’s neuronal networks, and understanding how ion channel dynamics mediate neural function. A challenge in defining such models is determining biophysically realistic channel distributions. Here, we present an efficient, highly parallel evolutionary algorithm for developing such models, named NeuroGPU-EA. NeuroGPU-EA uses CPUs and GPUs concurrently to simulate and evaluate neuron membrane potentials with respect to multiple stimuli. We demonstrate a logarithmic cost for scaling the stimuli used in the fitting procedure. NeuroGPU-EA outperforms the typically used CPU based evolutionary algorithm by a factor of 10 on a series of scaling benchmarks. We report observed performance bottlenecks and propose mitigation strategies. Finally, we also discuss the potential of this method for efficient simulation and evaluation of electrophysiological waveforms.
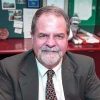
Dr. David Lowemann, M.Sc, Ph.D., is a co-founder of the Institute for the Future of Human Potential, where he leads the charge in pioneering Self-Enhancement Science for the Success of Society. With a keen interest in exploring the untapped potential of the human mind, Dr. Lowemann has dedicated his career to pushing the boundaries of human capabilities and understanding.
Armed with a Master of Science degree and a Ph.D. in his field, Dr. Lowemann has consistently been at the forefront of research and innovation, delving into ways to optimize human performance, cognition, and overall well-being. His work at the Institute revolves around a profound commitment to harnessing cutting-edge science and technology to help individuals lead more fulfilling and intelligent lives.
Dr. Lowemann’s influence extends to the educational platform BetterSmarter.me, where he shares his insights, findings, and personal development strategies with a broader audience. His ongoing mission is shaping the way we perceive and leverage the vast capacities of the human mind, offering invaluable contributions to society’s overall success and collective well-being.