Imagine if you could train a computer to read minds – well, almost! Scientists have developed a deep learning model that can predict the progression from mild cognitive impairment (MCI) to Alzheimer’s disease (AD) using structural MRI images of the brain. Just like studying a map to find your way, this computer-aided method analyzes 3D brain images to distinguish between cognitively normal individuals, those with MCI, and those with AD. The model, which was trained on a large database of MRI images, uses a convolutional neural network (CNN) to classify the brain scans. The researchers tested different CNN architectures, including ones trained on the ImageNet dataset, to achieve accurate classification. By identifying signs of AD early through this method, doctors can administer preventive medication and slow down the progression of the disease. Discover more about this exciting research by exploring the full article!
Alzheimer’s disease (AD) is an irreversible neurological disorder that affects the vast majority of dementia cases, leading patients to experience gradual memory loss and cognitive function decline. Despite the lack of a cure, early detection of Alzheimer’s disease permits the provision of preventive medication to slow the disease’s progression. The objective of this project is to develop a computer-aided method based on a deep learning model to distinguish Alzheimer’s disease (AD) from cognitively normal and its early stage, mild cognitive impairment (MCI), by just using structural MRI (sMRI). To attain this purpose, we proposed a multiclass classification method based on 3D T1-weight brain sMRI images from the ADNI database. Axial brain images were extracted from 3D MRI and fed into the convolutional neural network (CNN) for multiclass classification. Three separate models were tested: a CNN built from scratch, VGG-16, and ResNet-50. As a feature extractor, the VGG-16 and ResNet-50 convolutional bases trained on the ImageNet dataset were employed. To achieve classification, a new densely connected classifier was implemented on top of the convolutional bases.
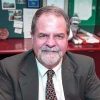
Dr. David Lowemann, M.Sc, Ph.D., is a co-founder of the Institute for the Future of Human Potential, where he leads the charge in pioneering Self-Enhancement Science for the Success of Society. With a keen interest in exploring the untapped potential of the human mind, Dr. Lowemann has dedicated his career to pushing the boundaries of human capabilities and understanding.
Armed with a Master of Science degree and a Ph.D. in his field, Dr. Lowemann has consistently been at the forefront of research and innovation, delving into ways to optimize human performance, cognition, and overall well-being. His work at the Institute revolves around a profound commitment to harnessing cutting-edge science and technology to help individuals lead more fulfilling and intelligent lives.
Dr. Lowemann’s influence extends to the educational platform BetterSmarter.me, where he shares his insights, findings, and personal development strategies with a broader audience. His ongoing mission is shaping the way we perceive and leverage the vast capacities of the human mind, offering invaluable contributions to society’s overall success and collective well-being.