Imagine a world where artificial neural networks not only recognize patterns but also actively generate predictions about what will happen next, just like our own brains! This fascinating research explores the possibility of building reflective spiking neural networks (SNNs) that can mimic the complex cognitive actions of our brain, taking advantage of their intrinsic dynamics. Unlike traditional artificial neural networks, which are driven by reflex-like responses, SNNs have the potential to make the leap towards true cognition. The development of SNNs faces challenges, especially in training, but recent insights into the high-dimensional nature of the brain offer promising solutions. Furthermore, memristive devices provide an exciting avenue for implementing neural networks by densely packing plastic synaptic contacts onto a single chip. These memristive systems can process analog information directly and offer energy-efficient in-memory and in-sensor computing. With memristive SNNs, we may witness a new era of cognitive and reflective computations beyond traditional neural networks. To dive deeper into this cutting-edge research, check out the full article!
The design of modern convolutional artificial neural networks (ANNs) composed of formal neurons copies the architecture of the visual cortex. Signals proceed through a hierarchy, where receptive fields become increasingly more complex and coding sparse. Nowadays, ANNs outperform humans in controlled pattern recognition tasks yet remain far behind in cognition. In part, it happens due to limited knowledge about the higher echelons of the brain hierarchy, where neurons actively generate predictions about what will happen next, i.e., the information processing jumps from reflex to reflection. In this study, we forecast that spiking neural networks (SNNs) can achieve the next qualitative leap. Reflective SNNs may take advantage of their intrinsic dynamics and mimic complex, not reflex-based, brain actions. They also enable a significant reduction in energy consumption. However, the training of SNNs is a challenging problem, strongly limiting their deployment. We then briefly overview new insights provided by the concept of a high-dimensional brain, which has been put forward to explain the potential power of single neurons in higher brain stations and deep SNN layers. Finally, we discuss the prospect of implementing neural networks in memristive systems. Such systems can densely pack on a chip 2D or 3D arrays of plastic synaptic contacts directly processing analog information. Thus, memristive devices are a good candidate for implementing in-memory and in-sensor computing. Then, memristive SNNs can diverge from the development of ANNs and build their niche, cognitive, or reflective computations.
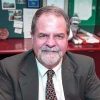
Dr. David Lowemann, M.Sc, Ph.D., is a co-founder of the Institute for the Future of Human Potential, where he leads the charge in pioneering Self-Enhancement Science for the Success of Society. With a keen interest in exploring the untapped potential of the human mind, Dr. Lowemann has dedicated his career to pushing the boundaries of human capabilities and understanding.
Armed with a Master of Science degree and a Ph.D. in his field, Dr. Lowemann has consistently been at the forefront of research and innovation, delving into ways to optimize human performance, cognition, and overall well-being. His work at the Institute revolves around a profound commitment to harnessing cutting-edge science and technology to help individuals lead more fulfilling and intelligent lives.
Dr. Lowemann’s influence extends to the educational platform BetterSmarter.me, where he shares his insights, findings, and personal development strategies with a broader audience. His ongoing mission is shaping the way we perceive and leverage the vast capacities of the human mind, offering invaluable contributions to society’s overall success and collective well-being.