Depression is like a dark cloud that hangs over many people, affecting their daily lives. Just as a skilled detective analyzes clues to solve a mystery, scientists have developed SparNet, a powerful tool for understanding major depressive disorder (MDD) using electroencephalogram (EEG) measurements. By combining advanced deep learning techniques with the unique abilities of SparNet, researchers were able to train the model to identify depression with an incredible accuracy of 94.37%! It’s like having a magnifying glass that can spot even the tiniest details in the brain. The SparNet model uses convolutional filters in different brain regions to learn about space-frequency features in EEG signals, allowing it to distinguish between depressive and normal control subjects. The results of the study not only validate the effectiveness of SparNet but also emphasize the importance of incorporating spatial and frequency domain information to successfully detect depression. If you’re curious to learn more about SparNet and how it can contribute to our understanding of depression, check out the research article!
Depression affects many people around the world today and is considered a global problem. Electroencephalogram (EEG) measurement is an appropriate way to understand the underlying mechanisms of major depressive disorder (MDD) to distinguish depression from normal control. With the development of deep learning methods, many researchers have adopted deep learning models to improve the classification accuracy of depression recognition. However, there are few studies on designing convolution filters for spatial and frequency domain feature learning in different brain regions. In this study, SparNet, a convolutional neural network composed of five parallel convolutional filters and the SENet, is proposed to learn EEG space-frequency domain characteristics and distinguish between depressive and normal control. The model is trained and tested by the cross-validation method of subject division. The results show that SparNet achieves a sensitivity of 95.07%, a specificity of 93.66%, and an accuracy of 94.37% in classification. Therefore, our results can conclude that the proposed SparNet model is effective in detecting depression using EEG signals. It also indicates that the combination of spatial information and frequency domain information is an effective way to identify patients with depression.
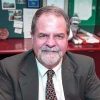
Dr. David Lowemann, M.Sc, Ph.D., is a co-founder of the Institute for the Future of Human Potential, where he leads the charge in pioneering Self-Enhancement Science for the Success of Society. With a keen interest in exploring the untapped potential of the human mind, Dr. Lowemann has dedicated his career to pushing the boundaries of human capabilities and understanding.
Armed with a Master of Science degree and a Ph.D. in his field, Dr. Lowemann has consistently been at the forefront of research and innovation, delving into ways to optimize human performance, cognition, and overall well-being. His work at the Institute revolves around a profound commitment to harnessing cutting-edge science and technology to help individuals lead more fulfilling and intelligent lives.
Dr. Lowemann’s influence extends to the educational platform BetterSmarter.me, where he shares his insights, findings, and personal development strategies with a broader audience. His ongoing mission is shaping the way we perceive and leverage the vast capacities of the human mind, offering invaluable contributions to society’s overall success and collective well-being.