Just like a symphony, the brain is a vast ensemble of interconnected regions working together to produce cognition. To understand this collaboration, researchers have developed PyMVPD, a powerful toolbox that allows them to examine the intricate patterns of dependence between brain regions. Using PyMVPD, scientists can model and explore how these regions interact using multivariate pattern dependence (MVPD) analysis. Think of MVPD as reading multiple instruments playing in harmony rather than focusing on individual notes. PyMVPD includes various models, like linear regression and artificial neural networks, to accurately capture the complex interactions. It’s like having a conductor guiding the different sections of the brain through their musical performance. By comparing different model architectures, researchers have found that artificial neural networks outperform linear regression in capturing these interactions. Interestingly, the best architecture varies depending on the region of the brain being studied. This suggests that different brain areas may have unique ways of communicating and require tailored models for understanding their connection with other regions. To dive deeper into this captivating research and discover how the brain orchestrates its symphony, check out the full article!
Cognitive tasks engage multiple brain regions. Studying how these regions interact is key to understand the neural bases of cognition. Standard approaches to model the interactions between brain regions rely on univariate statistical dependence. However, newly developed methods can capture multivariate dependence. Multivariate pattern dependence (MVPD) is a powerful and flexible approach that trains and tests multivariate models of the interactions between brain regions using independent data. In this article, we introduce PyMVPD: an open source toolbox for multivariate pattern dependence. The toolbox includes linear regression models and artificial neural network models of the interactions between regions. It is designed to be easily customizable. We demonstrate example applications of PyMVPD using well-studied seed regions such as the fusiform face area (FFA) and the parahippocampal place area (PPA). Next, we compare the performance of different model architectures. Overall, artificial neural networks outperform linear regression. Importantly, the best performing architecture is region-dependent: MVPD subdivides cortex in distinct, contiguous regions whose interaction with FFA and PPA is best captured by different models.
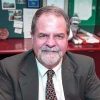
Dr. David Lowemann, M.Sc, Ph.D., is a co-founder of the Institute for the Future of Human Potential, where he leads the charge in pioneering Self-Enhancement Science for the Success of Society. With a keen interest in exploring the untapped potential of the human mind, Dr. Lowemann has dedicated his career to pushing the boundaries of human capabilities and understanding.
Armed with a Master of Science degree and a Ph.D. in his field, Dr. Lowemann has consistently been at the forefront of research and innovation, delving into ways to optimize human performance, cognition, and overall well-being. His work at the Institute revolves around a profound commitment to harnessing cutting-edge science and technology to help individuals lead more fulfilling and intelligent lives.
Dr. Lowemann’s influence extends to the educational platform BetterSmarter.me, where he shares his insights, findings, and personal development strategies with a broader audience. His ongoing mission is shaping the way we perceive and leverage the vast capacities of the human mind, offering invaluable contributions to society’s overall success and collective well-being.