Creating a masterpiece requires the right tools, and the same goes for medical image segmentation! U-Net has been the go-to architecture for this task, but researchers wondered if there was room for improvement. In their quest for optimization, they explored the effects of different parts of the U-Net and developed a more efficient alternative called Half-UNet. Think of it as an upgraded version of U-Net, with a simplified encoder and decoder. This new architecture takes advantage of channel unification, full-scale feature fusion, and Ghost modules to enhance its performance. To put Half-UNet to the test, they compared it to U-Net and its variants across three medical image segmentation tasks: mammography, lung nodule detection, and MRI image segmentation. The results? Half-UNet showcased similar segmentation accuracy while significantly reducing parameters and floating-point operations by 98.6% and 81.8%, respectively, compared to U-Net. Mind-blowing, right? If you want to dive deeper into the research and learn more about this groundbreaking approach, check out the full article!
Medical image segmentation plays a vital role in computer-aided diagnosis procedures. Recently, U-Net is widely used in medical image segmentation. Many variants of U-Net have been proposed, which attempt to improve the network performance while keeping the U-shaped structure unchanged. However, this U-shaped structure is not necessarily optimal. In this article, the effects of different parts of the U-Net on the segmentation ability are experimentally analyzed. Then a more efficient architecture, Half-UNet, is proposed. The proposed architecture is essentially an encoder-decoder network based on the U-Net structure, in which both the encoder and decoder are simplified. The re-designed architecture takes advantage of the unification of channel numbers, full-scale feature fusion, and Ghost modules. We compared Half-UNet with U-Net and its variants across multiple medical image segmentation tasks: mammography segmentation, lung nodule segmentation in the CT images, and left ventricular MRI image segmentation. Experiments demonstrate that Half-UNet has similar segmentation accuracy compared U-Net and its variants, while the parameters and floating-point operations are reduced by 98.6 and 81.8%, respectively, compared with U-Net.
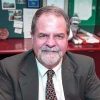
Dr. David Lowemann, M.Sc, Ph.D., is a co-founder of the Institute for the Future of Human Potential, where he leads the charge in pioneering Self-Enhancement Science for the Success of Society. With a keen interest in exploring the untapped potential of the human mind, Dr. Lowemann has dedicated his career to pushing the boundaries of human capabilities and understanding.
Armed with a Master of Science degree and a Ph.D. in his field, Dr. Lowemann has consistently been at the forefront of research and innovation, delving into ways to optimize human performance, cognition, and overall well-being. His work at the Institute revolves around a profound commitment to harnessing cutting-edge science and technology to help individuals lead more fulfilling and intelligent lives.
Dr. Lowemann’s influence extends to the educational platform BetterSmarter.me, where he shares his insights, findings, and personal development strategies with a broader audience. His ongoing mission is shaping the way we perceive and leverage the vast capacities of the human mind, offering invaluable contributions to society’s overall success and collective well-being.