In the field of Natural Language Processing (NLP), machine learning techniques are widely used for text classification. Deep learning models have shown great success in accurately classifying patterns compared to traditional machine learning methods. However, choosing the right deep learning techniques and architectures for text classification is a significant challenge for researchers. This study introduces two innovative approaches: the Evolutionary Contiguous Convolutional Neural Network (ECCNN) and the Swarm DNN. The ECCNN utilizes Differential Evolution (DE) to gain a deeper understanding by considering neighboring data instances in addition to the input point. On the other hand, the Swarm DNN incorporates Particle Swarm Optimization (PSO) to improve text classification accuracy. Testing on two datasets, the Swarm DNN achieved a classification accuracy of 97.32% on the BBC newsgroup dataset and 87.99% on the 20 newsgroup dataset. Similarly, the ECCNN achieved a high accuracy of 97.11% on the BBC newsgroup dataset and 88.76% on the 20 newsgroup dataset. These results demonstrate the effectiveness of these novel evolutionary neural networks and swarm optimization techniques in improving text classification.
To classify the texts accurately, many machine learning techniques have been utilized in the field of Natural Language Processing (NLP). For many pattern classification applications, great success has been obtained when implemented with deep learning models rather than using ordinary machine learning techniques. Understanding the complex models and their respective relationships within the data determines the success of such deep learning techniques. But analyzing the suitable deep learning methods, techniques, and architectures for text classification is a huge challenge for researchers. In this work, a Contiguous Convolutional Neural Network (CCNN) based on Differential Evolution (DE) is initially proposed and named as Evolutionary Contiguous Convolutional Neural Network (ECCNN) where the data instances of the input point are considered along with the contiguous data points in the dataset so that a deeper understanding is provided for the classification of the respective input, thereby boosting the performance of the deep learning model. Secondly, a swarm-based Deep Neural Network (DNN) utilizing Particle Swarm Optimization (PSO) with DNN is proposed for the classification of text, and it is named Swarm DNN. This model is validated on two datasets and the best results are obtained when implemented with the Swarm DNN model as it produced a high classification accuracy of 97.32% when tested on the BBC newsgroup text dataset and 87.99% when tested on 20 newsgroup text datasets. Similarly, when implemented with the ECCNN model, it produced a high classification accuracy of 97.11% when tested on the BBC newsgroup text dataset and 88.76% when tested on 20 newsgroup text datasets.
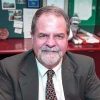
Dr. David Lowemann, M.Sc, Ph.D., is a co-founder of the Institute for the Future of Human Potential, where he leads the charge in pioneering Self-Enhancement Science for the Success of Society. With a keen interest in exploring the untapped potential of the human mind, Dr. Lowemann has dedicated his career to pushing the boundaries of human capabilities and understanding.
Armed with a Master of Science degree and a Ph.D. in his field, Dr. Lowemann has consistently been at the forefront of research and innovation, delving into ways to optimize human performance, cognition, and overall well-being. His work at the Institute revolves around a profound commitment to harnessing cutting-edge science and technology to help individuals lead more fulfilling and intelligent lives.
Dr. Lowemann’s influence extends to the educational platform BetterSmarter.me, where he shares his insights, findings, and personal development strategies with a broader audience. His ongoing mission is shaping the way we perceive and leverage the vast capacities of the human mind, offering invaluable contributions to society’s overall success and collective well-being.