In the world of brain-computer interface systems, detecting P300 (the main event-related potential) accurately is crucial. However, existing classification methods often struggle to maintain high accuracy when tested with new participants. To address this challenge, scientists developed a novel LR-CNN model that combines logistic regression and a convolutional neural network. This model consists of two parts: an LR-based memory model that learns individual participant features, and a CNN-based generalization model that identifies common features among participants. By utilizing these models, the researchers achieved a significant improvement in classification accuracy for new participants compared to traditional methods. The results of three sets of experiments demonstrated the effectiveness of the LR-CNN model in capturing individual differences and improving overall classification accuracy. This advancement holds great potential for enhancing the development of brain-computer interface systems and expanding their applications in various fields such as health care, identity recognition, and fatigue detection!
BackgroundElectroencephalogram (EEG)-based brain-computer interface (BCI) systems are widely utilized in various fields, including health care, intelligent assistance, identity recognition, emotion recognition, and fatigue detection. P300, the main event-related potential, is the primary component detected by EEG-based BCI systems. Existing algorithms for P300 classification in EEG data usually perform well when tested in a single participant, although they exhibit significant decreases in accuracy when tested in new participants. We attempted to address this lack of generalizability associated with existing classification methods using a novel convolutional neural network (CNN) model developed using logistic regression (LR).Materials and MethodsWe proposed an LR-CNN model comprising two parts: a combined LR-based memory model and a CNN-based generalization model. The LR-based memory model can learn the individual features of participants and addresses the decrease in accuracy caused by individual differences when applied to new participants. The CNN-based generalization model can learn the common features among participants, thereby reducing overall classification bias and improving overall classification accuracy.ResultsWe compared our method with existing, commonly used classification methods through three different sets of experiments. The experimental results indicated that our method could learn individual differences among participants. Compared with other commonly used classification methods, our method yielded a marked improvement (>90%) in classification among new participants.ConclusionThe accuracy of the proposed model in the face of new participants is better than that of existing, commonly used classification methods. Such improvements in cross-subject test accuracy will aid in the development of BCI systems.
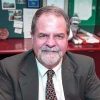
Dr. David Lowemann, M.Sc, Ph.D., is a co-founder of the Institute for the Future of Human Potential, where he leads the charge in pioneering Self-Enhancement Science for the Success of Society. With a keen interest in exploring the untapped potential of the human mind, Dr. Lowemann has dedicated his career to pushing the boundaries of human capabilities and understanding.
Armed with a Master of Science degree and a Ph.D. in his field, Dr. Lowemann has consistently been at the forefront of research and innovation, delving into ways to optimize human performance, cognition, and overall well-being. His work at the Institute revolves around a profound commitment to harnessing cutting-edge science and technology to help individuals lead more fulfilling and intelligent lives.
Dr. Lowemann’s influence extends to the educational platform BetterSmarter.me, where he shares his insights, findings, and personal development strategies with a broader audience. His ongoing mission is shaping the way we perceive and leverage the vast capacities of the human mind, offering invaluable contributions to society’s overall success and collective well-being.