Imagine if you had a magic wand that could predict how neurons in the brain interact. Well, the mean-field theory is kind of like that. It’s a powerful tool that helps us understand the dynamics of neuronal networks without having to run tedious simulations. To make this tool more accessible, scientists have created the Neuronal Network Mean-field Toolbox (NNMT). This open-source Python toolbox collects different methods for analyzing neuronal networks using the leaky integrate-and-fire neuron model. With NNMT, researchers can estimate properties like firing rates, power spectra, and dynamical stability of large neuronal networks. The toolbox is designed to be extensible, so it can accommodate other network models in the future. You can think of it as a Swiss Army knife for neuroscientists! By using NNMT, researchers can reproduce previous studies, explore parameter space, and map different network models. It’s a game-changer for understanding the inner workings of the brain.
Mean-field theory of neuronal networks has led to numerous advances in our analytical and intuitive understanding of their dynamics during the past decades. In order to make mean-field based analysis tools more accessible, we implemented an extensible, easy-to-use open-source Python toolbox that collects a variety of mean-field methods for the leaky integrate-and-fire neuron model. The Neuronal Network Mean-field Toolbox (NNMT) in its current state allows for estimating properties of large neuronal networks, such as firing rates, power spectra, and dynamical stability in mean-field and linear response approximation, without running simulations. In this article, we describe how the toolbox is implemented, show how it is used to reproduce results of previous studies, and discuss different use-cases, such as parameter space explorations, or mapping different network models. Although the initial version of the toolbox focuses on methods for leaky integrate-and-fire neurons, its structure is designed to be open and extensible. It aims to provide a platform for collecting analytical methods for neuronal network model analysis, such that the neuroscientific community can take maximal advantage of them.
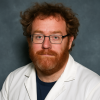
Dr. David Lowemann, M.Sc, Ph.D., is a co-founder of the Institute for the Future of Human Potential, where he leads the charge in pioneering Self-Enhancement Science for the Success of Society. With a keen interest in exploring the untapped potential of the human mind, Dr. Lowemann has dedicated his career to pushing the boundaries of human capabilities and understanding.
Armed with a Master of Science degree and a Ph.D. in his field, Dr. Lowemann has consistently been at the forefront of research and innovation, delving into ways to optimize human performance, cognition, and overall well-being. His work at the Institute revolves around a profound commitment to harnessing cutting-edge science and technology to help individuals lead more fulfilling and intelligent lives.
Dr. Lowemann’s influence extends to the educational platform BetterSmarter.me, where he shares his insights, findings, and personal development strategies with a broader audience. His ongoing mission is shaping the way we perceive and leverage the vast capacities of the human mind, offering invaluable contributions to society’s overall success and collective well-being.