Imagine you’re building a house. You need to know how different materials fit together and how they contribute to the overall structure. The same goes for constructing knowledge about cause and effect relationships. This paper explores how children develop abstract domain-general knowledge that helps them understand specific causal relationships. It specifically focuses on the role of analytic knowledge, which breaks down observed outcomes into constituent causes. The researchers conducted two experiments with preschool-age children to test their hypothesis. The findings suggest that even young children possess an innate understanding of causal invariance, using it to navigate and generalize across different contexts involving binary cause and effect variables. This highlights the importance of analytic knowledge in shaping our empirical understanding of causality. By ruling out alternative explanations and biases, this research sheds light on the rational way in which we comprehend cause and effect relationships. If you’re curious to learn more about how children develop their understanding of causality, click the link below to explore the full article!
Abstract
The present paper examines a type of abstract domain-general knowledge required for the process of constructing useable domain-specific causal knowledge, the evident goal of causal learning. It tests the hypothesis that analytic knowledge of causal-invariance decomposition functions is essential for this process. Such knowledge specifies the decomposition of an observed outcome into contributions from constituent causes under the default assumption that the empirical knowledge acquired is invariant across contextual/background causes. The paper reports two psychological experiments (and replication studies) with pre-school-age children on generalization across contexts involving binary cause and effect variables. The critical role of causal invariance for constructing useable causal knowledge predicts that even young children should (tacitly) use the causal-invariance decomposition function for such variables rather than a non-causal-invariance decomposition function common in statistical practice in research involving binary outcomes. The findings support the rational shaping of empirical causal knowledge by the causal-invariance constraint, ruling out alternative explanations in terms of non-causal-invariance decomposition functions, heuristics, and biases. For the same causal structure involving candidate causes and outcomes that are binary variables with a “present” value and an “absent” value, the paper argues against the possibility of multiple rational characterizations of the “sameness of causal influence” that justifies generalization across contexts.
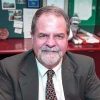
Dr. David Lowemann, M.Sc, Ph.D., is a co-founder of the Institute for the Future of Human Potential, where he leads the charge in pioneering Self-Enhancement Science for the Success of Society. With a keen interest in exploring the untapped potential of the human mind, Dr. Lowemann has dedicated his career to pushing the boundaries of human capabilities and understanding.
Armed with a Master of Science degree and a Ph.D. in his field, Dr. Lowemann has consistently been at the forefront of research and innovation, delving into ways to optimize human performance, cognition, and overall well-being. His work at the Institute revolves around a profound commitment to harnessing cutting-edge science and technology to help individuals lead more fulfilling and intelligent lives.
Dr. Lowemann’s influence extends to the educational platform BetterSmarter.me, where he shares his insights, findings, and personal development strategies with a broader audience. His ongoing mission is shaping the way we perceive and leverage the vast capacities of the human mind, offering invaluable contributions to society’s overall success and collective well-being.