Imagine your brain is like a beautiful symphony, with each instrument representing different regions working together in perfect harmony. But what happens when this symphony starts to lose its rhythm? That’s Alzheimer’s disease, a neurological disorder that disrupts the brain’s cognitive function and behavior. Detecting and treating Alzheimer’s early is critical, and scientists have found a powerful tool: electroencephalography (EEG) signals. These signals, which are non-invasive and measure electrical activity in the brain, can provide valuable insights into the presence of Alzheimer’s disease. But analyzing these signals can be challenging when using single-channel EEG data. That’s why researchers developed a new algorithm called No-threshold Recurrence Plot Convolution Network (NRPCN), which analyzes multiple channels simultaneously and creates a two-dimensional representation of the EEG signals. This breakthrough allows for a more comprehensive understanding of the disease. Furthermore, the algorithm combines clinical features and imaging data to enhance its accuracy. The results of the experiments have shown that this algorithm performs exceptionally well and is robust in detecting Alzheimer’s disease. To learn more about this groundbreaking research and its potential implications for early Alzheimer’s detection, check out the full article!
Alzheimer’s disease is a neurological disorder characterized by progressive cognitive dysfunction and behavioral impairment that occurs in old. Early diagnosis and treatment of Alzheimer’s disease is great significance. Electroencephalography (EEG) signals can be used to detect Alzheimer’s disease due to its non-invasive advantage. To solve the problem of insufficient analysis by single-channel EEG signal, we analyze the relationship between multiple channels and build PLV framework. To solve the problem of insufficient representation of 1D signal, a threshold-free recursive plot convolution network was constructed to realize 2D representation. To solve the problem of insufficient EEG signal characterization, a fusion algorithm of clinical features and imaging features was proposed to detect Alzheimer’s disease. Experimental results show that the algorithm has good performance and robustness.
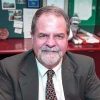
Dr. David Lowemann, M.Sc, Ph.D., is a co-founder of the Institute for the Future of Human Potential, where he leads the charge in pioneering Self-Enhancement Science for the Success of Society. With a keen interest in exploring the untapped potential of the human mind, Dr. Lowemann has dedicated his career to pushing the boundaries of human capabilities and understanding.
Armed with a Master of Science degree and a Ph.D. in his field, Dr. Lowemann has consistently been at the forefront of research and innovation, delving into ways to optimize human performance, cognition, and overall well-being. His work at the Institute revolves around a profound commitment to harnessing cutting-edge science and technology to help individuals lead more fulfilling and intelligent lives.
Dr. Lowemann’s influence extends to the educational platform BetterSmarter.me, where he shares his insights, findings, and personal development strategies with a broader audience. His ongoing mission is shaping the way we perceive and leverage the vast capacities of the human mind, offering invaluable contributions to society’s overall success and collective well-being.