Imagine you have a superhero who is not only able to identify villains but also predict their future plans. That’s what researchers are working on in the field of Alzheimer’s disease (AD) diagnosis. Typically, algorithms focus on either classifying patients or predicting their cognitive scores. However, a new study takes it a step further by proposing a multitask deep neural network that tackles both tasks simultaneously. By combining multiple types of data, such as images and biomarkers, the network aims to achieve the highest accuracy in classifying patients and predicting their future cognitive decline.
The key to this superhero-like algorithm is modality fusion, kernelization, and tensorization. Think of it as merging different superpowers into one ultimate force! The researchers tested various multimodality scenarios and exploited the complementary aspects of the different data types. The results were promising, with an overall classification accuracy of around 66.85%, beating other state-of-the-art techniques in the field.
Interestingly, the study also found that optimizing hyperparameters for one task might not be beneficial for the other task. It’s like finding the right balance between speed and accuracy—pushing too hard on one might compromise the performance of the other. These findings shed light on the delicate trade-offs involved in designing efficient multitask algorithms for AD diagnosis.
If you’re intrigued by this exciting research, be sure to check out the full article for more details!
With the advances in machine learning for the diagnosis of Alzheimer’s disease (AD), most studies have focused on either identifying the subject’s status through classification algorithms or on predicting their cognitive scores through regression methods, neglecting the potential association between these two tasks. Motivated by the need to enhance the prospects for early diagnosis along with the ability to predict future disease states, this study proposes a deep neural network based on modality fusion, kernelization, and tensorization that perform multiclass classification and longitudinal regression simultaneously within a unified multitask framework. This relationship between multiclass classification and longitudinal regression is found to boost the efficacy of the final model in dealing with both tasks. Different multimodality scenarios are investigated, and complementary aspects of the multimodal features are exploited to simultaneously delineate the subject’s label and predict related cognitive scores at future timepoints using baseline data. The main intent in this multitask framework is to consolidate the highest accuracy possible in terms of precision, sensitivity, F1 score, and area under the curve (AUC) in the multiclass classification task while maintaining the highest similarity in the MMSE score as measured through the correlation coefficient and the RMSE for all time points under the prediction task, with both tasks, run simultaneously under the same set of hyperparameters. The overall accuracy for multiclass classification of the proposed KTMnet method is 66.85 ± 3.77. The prediction results show an average RMSE of 2.32 ± 0.52 and a correlation of 0.71 ± 5.98 for predicting MMSE throughout the time points. These results are compared to state-of-the-art techniques reported in the literature. A discovery from the multitasking of this consolidated machine learning framework is that a set of hyperparameters that optimize the prediction results may not necessarily be the same as those that would optimize the multiclass classification. In other words, there is a breakpoint beyond which enhancing further the results of one process could lead to the downgrading in accuracy for the other.
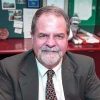
Dr. David Lowemann, M.Sc, Ph.D., is a co-founder of the Institute for the Future of Human Potential, where he leads the charge in pioneering Self-Enhancement Science for the Success of Society. With a keen interest in exploring the untapped potential of the human mind, Dr. Lowemann has dedicated his career to pushing the boundaries of human capabilities and understanding.
Armed with a Master of Science degree and a Ph.D. in his field, Dr. Lowemann has consistently been at the forefront of research and innovation, delving into ways to optimize human performance, cognition, and overall well-being. His work at the Institute revolves around a profound commitment to harnessing cutting-edge science and technology to help individuals lead more fulfilling and intelligent lives.
Dr. Lowemann’s influence extends to the educational platform BetterSmarter.me, where he shares his insights, findings, and personal development strategies with a broader audience. His ongoing mission is shaping the way we perceive and leverage the vast capacities of the human mind, offering invaluable contributions to society’s overall success and collective well-being.