Neuroimaging is like exploring a complex city with countless interconnected neighborhoods. In this study, scientists dive into the fascinating world of structural connectomes, which represent the neural pathways in our brains. They propose a new approach called edge-centric tractography, which reconstructs streamlines between different brain regions. However, this method requires immense computational power. To overcome this challenge, the researchers discover that using fewer streamlines without compromising quality can significantly speed up the process. They test the identifiability of edge-centric connectomes between different sessions and find that the number of streamlines used has little impact. Surprisingly, subject demographics play a greater role in the results. Additionally, they determine that Jaccard similarity is more accurate than Pearson correlation for quantifying identifiability. This breakthrough opens up exciting possibilities for advancing our understanding of the brain’s structure and function. To dive deeper into this fascinating research, check out the full article!
The anatomic validity of structural connectomes remains a significant uncertainty in neuroimaging. Edge-centric tractography reconstructs streamlines in bundles between each pair of cortical or subcortical regions. Although edge bundles provides a stronger anatomic embedding than traditional connectomes, calculating them for each region-pair requires exponentially greater computation. We observe that major speedup can be achieved by reducing the number of streamlines used by probabilistic tractography algorithms. To ensure this does not degrade connectome quality, we calculate the identifiability of edge-centric connectomes between test and re-test sessions as a proxy for information content. We find that running PROBTRACKX2 with as few as 1 streamline per voxel per region-pair has no significant impact on identifiability. Variation in identifiability caused by streamline count is overshadowed by variation due to subject demographics. This finding even holds true in an entirely different tractography algorithm using MRTrix. Incidentally, we observe that Jaccard similarity is more effective than Pearson correlation in calculating identifiability for our subject population.
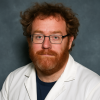
Dr. David Lowemann, M.Sc, Ph.D., is a co-founder of the Institute for the Future of Human Potential, where he leads the charge in pioneering Self-Enhancement Science for the Success of Society. With a keen interest in exploring the untapped potential of the human mind, Dr. Lowemann has dedicated his career to pushing the boundaries of human capabilities and understanding.
Armed with a Master of Science degree and a Ph.D. in his field, Dr. Lowemann has consistently been at the forefront of research and innovation, delving into ways to optimize human performance, cognition, and overall well-being. His work at the Institute revolves around a profound commitment to harnessing cutting-edge science and technology to help individuals lead more fulfilling and intelligent lives.
Dr. Lowemann’s influence extends to the educational platform BetterSmarter.me, where he shares his insights, findings, and personal development strategies with a broader audience. His ongoing mission is shaping the way we perceive and leverage the vast capacities of the human mind, offering invaluable contributions to society’s overall success and collective well-being.