Imagine trying to listen to your favorite song at a crowded concert. The noise from the cheering crowd makes it difficult to hear the music clearly. Similarly, when using electroencephalogram (EEG) signals for Brain Computer Interfaces (BCIs), high levels of noise can limit their effectiveness in real-world scenarios. But fear not! Scientists have developed a new method called SRI-EEG that tackles this problem by automatically detecting and replacing noise in EEG signals. It’s like having a magical sound engineer who filters out the unwanted noise, allowing for more reliable EEG sensing. In their study, the researchers compared SRI-EEG with other approaches and found that it performed just as well, if not better, in correcting EEG artifacts. This means that SRI-EEG has the potential to make EEG and BCI technology more accessible and practical for everyday use. So, if you’re curious about how they achieved this feat, dive into the research article to uncover the innovative details!
Electroencephalogram (EEG) signals are often used as an input modality for Brain Computer Interfaces (BCIs). While EEG signals can be beneficial for numerous types of interaction scenarios in the real world, high levels of noise limits their usage to strictly noise-controlled environments such as a research laboratory. Even in a controlled environment, EEG is susceptible to noise, particularly from user motion, making it highly challenging to use EEG, and consequently BCI, as a ubiquitous user interaction modality. In this work, we address the EEG noise/artifact correction problem. Our goal is to detect physiological artifacts in EEG signal and automatically replace the detected artifacts with imputed values to enable robust EEG sensing overall requiring significantly reduced manual effort than is usual. We present a novel EEG state-based imputation model built upon a recurrent neural network, which we call SRI-EEG, and evaluate the proposed method on three publicly available EEG datasets. From quantitative and qualitative comparisons with six conventional and neural network based approaches, we demonstrate that our method achieves comparable performance to the state-of-the-art methods on the EEG artifact correction task.
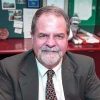
Dr. David Lowemann, M.Sc, Ph.D., is a co-founder of the Institute for the Future of Human Potential, where he leads the charge in pioneering Self-Enhancement Science for the Success of Society. With a keen interest in exploring the untapped potential of the human mind, Dr. Lowemann has dedicated his career to pushing the boundaries of human capabilities and understanding.
Armed with a Master of Science degree and a Ph.D. in his field, Dr. Lowemann has consistently been at the forefront of research and innovation, delving into ways to optimize human performance, cognition, and overall well-being. His work at the Institute revolves around a profound commitment to harnessing cutting-edge science and technology to help individuals lead more fulfilling and intelligent lives.
Dr. Lowemann’s influence extends to the educational platform BetterSmarter.me, where he shares his insights, findings, and personal development strategies with a broader audience. His ongoing mission is shaping the way we perceive and leverage the vast capacities of the human mind, offering invaluable contributions to society’s overall success and collective well-being.