In decision making, we often focus on only a few options out of many. This behavior may seem hasty, but it can actually be the smartest move when we have limited cognitive resources. Researchers have explored how to best allocate our limited sampling time to determine the most profitable option. They used accumulator models, which simulate how evidence accumulates for different choices. The study found that sampling capacity increases with available time and the distinguishability of options. The optimal allocation of time undergoes a dramatic shift based on capacity. For small capacities, evenly allocating time to five options while ignoring others is ideal, regardless of the rewards distribution. On the other hand, for larger capacities, the number of sampled accumulators grows in a sublinear fashion, following a power law. Interestingly, allocating equal time to each sampled accumulator is more effective than uneven allocations. This research sheds light on the tradeoffs involved in multialternative decision making and emphasizes the importance of limited resources and environmental variability. So next time you’re faced with numerous options, take a cue from science and focus on a handful that offers the most promise! To dive deeper, check out the full article.
Optimal Sampling Allocation in Decision Making Models
Abstract
When facing many options, we narrow down our focus to very few of them. Although behaviors like this can be a sign of heuristics, they can actually be optimal under limited cognitive resources. Here, we study the problem of how to optimally allocate limited sampling time to multiple options, modeled as accumulators of noisy evidence, to determine the most profitable one. We show that the effective sampling capacity of an agent increases with both available time and the discriminability of the options, and optimal policies undergo a sharp transition as a function of it. For small capacity, it is best to allocate time evenly to exactly five options and to ignore all the others, regardless of the prior distribution of rewards. For large capacities, the optimal number of sampled accumulators grows sublinearly, closely following a power law as a function of capacity for a wide variety of priors. We find that allocating equal times to the sampled accumulators is better than using uneven time allocations. Our work highlights that multialternative decisions are endowed with breadth–depth tradeoffs, demonstrates how their optimal solutions depend on the amount of limited resources and the variability of the environment, and shows that narrowing down to a handful of options is always optimal for small capacities.
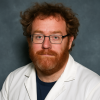
Dr. David Lowemann, M.Sc, Ph.D., is a co-founder of the Institute for the Future of Human Potential, where he leads the charge in pioneering Self-Enhancement Science for the Success of Society. With a keen interest in exploring the untapped potential of the human mind, Dr. Lowemann has dedicated his career to pushing the boundaries of human capabilities and understanding.
Armed with a Master of Science degree and a Ph.D. in his field, Dr. Lowemann has consistently been at the forefront of research and innovation, delving into ways to optimize human performance, cognition, and overall well-being. His work at the Institute revolves around a profound commitment to harnessing cutting-edge science and technology to help individuals lead more fulfilling and intelligent lives.
Dr. Lowemann’s influence extends to the educational platform BetterSmarter.me, where he shares his insights, findings, and personal development strategies with a broader audience. His ongoing mission is shaping the way we perceive and leverage the vast capacities of the human mind, offering invaluable contributions to society’s overall success and collective well-being.