Imagine your brain as a complex network of highways and byways. Now, imagine that some of those routes start to deteriorate, causing cognitive decline. That’s what happens in Alzheimer’s Disease (AD) and mild cognitive impairment (MCI). But how can we pinpoint the specific areas of the brain that are affected? Well, scientists have turned to machine learning for help! In a recent study, researchers used a machine learning tool called Hollow Tree Super (HoTS) to analyze brain scans of AD and MCI patients. By examining functional connectivity in the brain, they were able to predict performance on neuropsychological tests. The results revealed important brain regions associated with cognitive deficits and identified networks like the Central Executive Network and Default Mode Network as potential diagnostic targets. This innovative approach may improve early diagnosis and treatment selection for AD and MCI patients. If you’re curious about the intricate inner workings of the brain and how machine learning can unlock its secrets, check out the full article!
ObjectiveAlzheimer’s Disease (AD) is a progressive condition characterized by cognitive decline. AD is often preceded by mild cognitive impairment (MCI), though the diagnosis of both conditions remains a challenge. Early diagnosis of AD, and prediction of MCI progression require data-driven approaches to improve patient selection for treatment. We used a machine learning tool to predict performance in neuropsychological tests in AD and MCI based on functional connectivity using a whole-brain connectome, in an attempt to identify network substrates of cognitive deficits in AD.MethodsNeuropsychological tests, baseline anatomical T1 magnetic resonance imaging (MRI), resting-state functional MRI, and diffusion weighted imaging scans were obtained from 149 MCI, and 85 AD patients; and 140 cognitively unimpaired geriatric participants. A novel machine learning tool, Hollow Tree Super (HoTS) was utilized to extract feature importance from each machine learning model to identify brain regions that were associated with deficit and absence of deficit for 11 neuropsychological tests.Results11 models attained an area under the receiver operating curve (AUC-ROC) greater than 0.65, while five models had an AUC-ROC ≥ 0.7. 20 parcels of the Human Connectome Project Multimodal Parcelation Atlas matched to poor performance in at least two neuropsychological tests, while 14 parcels were associated with good performance in at least two tests. At a network level, most parcels predictive of both presence and absence of deficit were affiliated with the Central Executive Network, Default Mode Network, and the Sensorimotor Networks. Segregating predictors by the cognitive domain associated with each test revealed areas of coherent overlap between cognitive domains, with the parcels providing possible markers to screen for cognitive impairment.ConclusionApproaches such as ours which incorporate whole-brain functional connectivity and harness feature importance in machine learning models may aid in identifying diagnostic and therapeutic targets in AD.
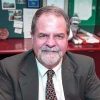
Dr. David Lowemann, M.Sc, Ph.D., is a co-founder of the Institute for the Future of Human Potential, where he leads the charge in pioneering Self-Enhancement Science for the Success of Society. With a keen interest in exploring the untapped potential of the human mind, Dr. Lowemann has dedicated his career to pushing the boundaries of human capabilities and understanding.
Armed with a Master of Science degree and a Ph.D. in his field, Dr. Lowemann has consistently been at the forefront of research and innovation, delving into ways to optimize human performance, cognition, and overall well-being. His work at the Institute revolves around a profound commitment to harnessing cutting-edge science and technology to help individuals lead more fulfilling and intelligent lives.
Dr. Lowemann’s influence extends to the educational platform BetterSmarter.me, where he shares his insights, findings, and personal development strategies with a broader audience. His ongoing mission is shaping the way we perceive and leverage the vast capacities of the human mind, offering invaluable contributions to society’s overall success and collective well-being.