Imagine trying to solve a puzzle, but you can only see a small portion of each piece at a time. That’s how convolutional neural networks (CNNs) have traditionally tackled medical image segmentation tasks. But now, researchers have developed LLRHNet, a new approach that combines the best of both worlds – local and long-range features. It’s like having a magnifying glass to examine the intricate details and a telescope to capture the big picture. By using an encoder-decoder architecture and incorporating an iterative aggregation mechanism and transformer technology, LLRHNet can gather information from different layers and fuse it together for more accurate segmentation. Not only does it excel in isolating target tissues, but it also shines in multiple lesions scenarios, as demonstrated by its outstanding performance in liver-related and stroke/white matter hyperintensity segmentation datasets. If you’re curious to dive deeper into this cutting-edge model, check out the full article!
The encoder-decoder-based deep convolutional neural networks (CNNs) have made great improvements in medical image segmentation tasks. However, due to the inherent locality of convolution, CNNs generally are demonstrated to have limitations in obtaining features across layers and long-range features from the medical image. In this study, we develop a local-long range hybrid features network (LLRHNet), which inherits the merits of the iterative aggregation mechanism and the transformer technology, as a medical image segmentation model. LLRHNet adopts encoder-decoder architecture as the backbone which iteratively aggregates the projection and up-sampling to fuse local low-high resolution features across isolated layers. The transformer adopts the multi-head self-attention mechanism to extract long-range features from the tokenized image patches and fuses these features with the local-range features extracted by down-sampling operation in the backbone network. These hybrid features are used to assist the cascaded up-sampling operations to local the position of the target tissues. LLRHNet is evaluated on two multiple lesions medical image data sets, including a public liver-related segmentation data set (3DIRCADb) and an in-house stroke and white matter hyperintensity (SWMH) segmentation data set. Experimental results denote that LLRHNet achieves state-of-the-art performance on both data sets.
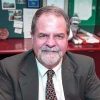
Dr. David Lowemann, M.Sc, Ph.D., is a co-founder of the Institute for the Future of Human Potential, where he leads the charge in pioneering Self-Enhancement Science for the Success of Society. With a keen interest in exploring the untapped potential of the human mind, Dr. Lowemann has dedicated his career to pushing the boundaries of human capabilities and understanding.
Armed with a Master of Science degree and a Ph.D. in his field, Dr. Lowemann has consistently been at the forefront of research and innovation, delving into ways to optimize human performance, cognition, and overall well-being. His work at the Institute revolves around a profound commitment to harnessing cutting-edge science and technology to help individuals lead more fulfilling and intelligent lives.
Dr. Lowemann’s influence extends to the educational platform BetterSmarter.me, where he shares his insights, findings, and personal development strategies with a broader audience. His ongoing mission is shaping the way we perceive and leverage the vast capacities of the human mind, offering invaluable contributions to society’s overall success and collective well-being.