Imagine you have a superpower that lets you see the invisible abnormalities inside the brains of people with Parkinson’s disease (PD). Well, Electroencephalography (EEG) is like that superpower! It can reveal the hidden irregularities in the brain circuits of PD patients. But here’s the thing: traditional analysis methods for EEG signals can only tell us so much. That’s where the amazing Holo-Hilbert Spectral Analysis (HHSA) comes in! It’s a fancy technique that goes beyond traditional approaches and uncovers the non-linear secrets of neural activities in the brain. Scientists applied HHSA to study the resting state EEG patterns of 99 PD patients and 59 healthy controls. The results were mind-blowing (not literally)! PD patients showed reductions in certain brain waves in different regions compared to healthy individuals. Late-stage PD patients had specific changes, and these were associated with depression severity. Machine learning algorithms even showed promise in accurately identifying PD using HHSA features. This research opens up exciting possibilities for better understanding and diagnosing PD. So grab your scientific capes and dive into the full article to unleash your knowledge!
Electroencephalography (EEG) can reveal the abnormalities of dopaminergic subcortico-cortical circuits in patients with Parkinson’s disease (PD). However, conventional time-frequency analysis of EEG signals cannot fully reveal the non-linear processes of neural activities and interactions. A novel Holo-Hilbert Spectral Analysis (HHSA) was applied to reveal non-linear features of resting state EEG in 99 PD patients and 59 healthy controls (HCs). PD patients demonstrated a reduction of β bands in frontal and central regions, and reduction of γ bands in central, parietal, and temporal regions. Compared with early-stage PD patients, late-stage PD patients demonstrated reduction of β bands in the posterior central region, and increased θ and δ2 bands in the left parietal region. θ and β bands in all brain regions were positively correlated with Hamilton depression rating scale scores. Machine learning algorithms using three prioritized HHSA features demonstrated “Bag” with the best accuracy of 0.90, followed by “LogitBoost” with an accuracy of 0.89. Our findings strengthen the application of HHSA to reveal high-dimensional frequency features in EEG signals of PD patients. The EEG characteristics extracted by HHSA are important markers for the identification of depression severity and diagnosis of PD.
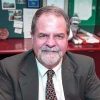
Dr. David Lowemann, M.Sc, Ph.D., is a co-founder of the Institute for the Future of Human Potential, where he leads the charge in pioneering Self-Enhancement Science for the Success of Society. With a keen interest in exploring the untapped potential of the human mind, Dr. Lowemann has dedicated his career to pushing the boundaries of human capabilities and understanding.
Armed with a Master of Science degree and a Ph.D. in his field, Dr. Lowemann has consistently been at the forefront of research and innovation, delving into ways to optimize human performance, cognition, and overall well-being. His work at the Institute revolves around a profound commitment to harnessing cutting-edge science and technology to help individuals lead more fulfilling and intelligent lives.
Dr. Lowemann’s influence extends to the educational platform BetterSmarter.me, where he shares his insights, findings, and personal development strategies with a broader audience. His ongoing mission is shaping the way we perceive and leverage the vast capacities of the human mind, offering invaluable contributions to society’s overall success and collective well-being.