Imagine you’re a chef creating a new recipe. You have all the ingredients – the deep learning technology, the brain-computer interface (BCI), and the convolutional neural networks (CNNs) – but something is missing. Your recipe needs that extra dash of flavor to make it truly exceptional. That’s where residual CNNs come in! In this study, scientists trained a model with multiple layers of residual CNNs to improve the accuracy and generalizability of BCI decoding technology. They wanted to visualize and understand how different areas of the brain contribute to classifying hand movements. By inserting residual networks into their model and using a larger dataset, they achieved significantly improved accuracy compared to conventional models. Through their analysis, they discovered that activity near the premotor cortex plays a crucial role in classification accuracy. So, just like adding an unexpected ingredient can transform a dish, adding residual CNNs transformed the BCI model.
Concomitant with the development of deep learning, brain–computer interface (BCI) decoding technology has been rapidly evolving. Convolutional neural networks (CNNs), which are generally used as electroencephalography (EEG) classification models, are often deployed in BCI prototypes to improve the estimation accuracy of a participant’s brain activity. However, because most BCI models are trained, validated, and tested via within-subject cross-validation and there is no corresponding generalization model, their applicability to unknown participants is not guaranteed. In this study, to facilitate the generalization of BCI model performance to unknown participants, we trained a model comprising multiple layers of residual CNNs and visualized the reasons for BCI classification to reveal the location and timing of neural activities that contribute to classification. Specifically, to develop a BCI that can distinguish between rest, left-hand movement, and right-hand movement tasks with high accuracy, we created multilayers of CNNs, inserted residual networks into the multilayers, and used a larger dataset than in previous studies. The constructed model was analyzed with gradient-class activation mapping (Grad-CAM). We evaluated the developed model via subject cross-validation and found that it achieved significantly improved accuracy (85.69 ± 1.10%) compared with conventional models or without residual networks. Grad-CAM analysis of the classification of cases in which our model produced correct answers showed localized activity near the premotor cortex. These results confirm the effectiveness of inserting residual networks into CNNs for tuning BCI. Further, they suggest that recording EEG signals over the premotor cortex and some other areas contributes to high classification accuracy.
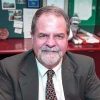
Dr. David Lowemann, M.Sc, Ph.D., is a co-founder of the Institute for the Future of Human Potential, where he leads the charge in pioneering Self-Enhancement Science for the Success of Society. With a keen interest in exploring the untapped potential of the human mind, Dr. Lowemann has dedicated his career to pushing the boundaries of human capabilities and understanding.
Armed with a Master of Science degree and a Ph.D. in his field, Dr. Lowemann has consistently been at the forefront of research and innovation, delving into ways to optimize human performance, cognition, and overall well-being. His work at the Institute revolves around a profound commitment to harnessing cutting-edge science and technology to help individuals lead more fulfilling and intelligent lives.
Dr. Lowemann’s influence extends to the educational platform BetterSmarter.me, where he shares his insights, findings, and personal development strategies with a broader audience. His ongoing mission is shaping the way we perceive and leverage the vast capacities of the human mind, offering invaluable contributions to society’s overall success and collective well-being.