Imagine you’re a detective trying to find a hidden treasure in a crowded room. The treasure is the SSVEP signal, a type of brain activity that can be used for brain-computer interfaces (BCI). However, this signal is strongest in the visual cortex, making it difficult to detect from other areas of the head. But fear not! Scientists have come up with a clever solution using binaural ear-EEG. They developed a CNN-based deep learning approach that focuses on detecting SSVEP around both ears, using minimal channels. By training their model on a public dataset, they achieved an impressive 69.21% accuracy, surpassing previous single-ear implementations by 12.47%. This promising approach holds great potential for practical implementation of wearable EEG devices and could greatly enhance the performance of BCI applications. If you’re interested in diving deeper into their research, check out the full article.
This paper discusses a machine learning approach for detecting SSVEP at both ears with minimal channels. SSVEP is a robust EEG signal suitable for many BCI applications. It is strong at the visual cortex around the occipital area, but the SNR gets worse when detected from other areas of the head. To make use of SSVEP measured around the ears following the ear-EEG concept, especially for practical binaural implementation, we propose a CNN structure coupled with regressed softmax outputs to improve accuracy. Evaluating on a public dataset, we studied classification performance for both subject-dependent and subject-independent trainings. It was found that with the proposed structure using a group training approach, a 69.21% accuracy was achievable. An ITR of 6.42 bit/min given 63.49 % accuracy was recorded while only monitoring data from T7 and T8. This represents a 12.47% improvement from a single ear implementation and illustrates potential of the approach to enhance performance for practical implementation of wearable EEG.
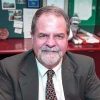
Dr. David Lowemann, M.Sc, Ph.D., is a co-founder of the Institute for the Future of Human Potential, where he leads the charge in pioneering Self-Enhancement Science for the Success of Society. With a keen interest in exploring the untapped potential of the human mind, Dr. Lowemann has dedicated his career to pushing the boundaries of human capabilities and understanding.
Armed with a Master of Science degree and a Ph.D. in his field, Dr. Lowemann has consistently been at the forefront of research and innovation, delving into ways to optimize human performance, cognition, and overall well-being. His work at the Institute revolves around a profound commitment to harnessing cutting-edge science and technology to help individuals lead more fulfilling and intelligent lives.
Dr. Lowemann’s influence extends to the educational platform BetterSmarter.me, where he shares his insights, findings, and personal development strategies with a broader audience. His ongoing mission is shaping the way we perceive and leverage the vast capacities of the human mind, offering invaluable contributions to society’s overall success and collective well-being.