Imagine you have a treasure map, but it’s written in a secret code. You can see it, but you can’t understand it. That’s the challenge scientists faced when using convolutional neural networks (CNNs) to analyze raw electroencephalography (EEG) data. The problem? CNNs are great at learning patterns in EEG data, but they don’t explain what those patterns mean. So, researchers developed a new approach that combines the power of CNNs with interpretability. It’s like having a translator for the treasure map! This approach uses model visualization to make the CNN architecture more understandable and then applies a series of explainability methods. By evaluating specific clusters of filters and the contributions of identified waveforms and spectra, scientists can better understand the important features in resting-state EEG data. In fact, when applied to automated sleep stage classification, this approach aligns closely with established clinical guidelines. This groundbreaking method is the first to systematically evaluate both waveform and spectral feature importance in CNNs trained on raw EEG data. So why wait? Explore the research now and unlock the hidden secrets of EEG!
In recent years, the use of convolutional neural networks (CNNs) for raw resting-state electroencephalography (EEG) analysis has grown increasingly common. However, relative to earlier machine learning and deep learning methods with manually extracted features, CNNs for raw EEG analysis present unique problems for explainability. As such, a growing group of methods have been developed that provide insight into the spectral features learned by CNNs. However, spectral power is not the only important form of information within EEG, and the capacity to understand the roles of specific multispectral waveforms identified by CNNs could be very helpful. In this study, we present a novel model visualization-based approach that adapts the traditional CNN architecture to increase interpretability and combines that inherent interpretability with a systematic evaluation of the model via a series of novel explainability methods. Our approach evaluates the importance of spectrally distinct first-layer clusters of filters before examining the contributions of identified waveforms and spectra to cluster importance. We evaluate our approach within the context of automated sleep stage classification and find that, for the most part, our explainability results are highly consistent with clinical guidelines. Our approach is the first to systematically evaluate both waveform and spectral feature importance in CNNs trained on resting-state EEG data.
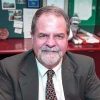
Dr. David Lowemann, M.Sc, Ph.D., is a co-founder of the Institute for the Future of Human Potential, where he leads the charge in pioneering Self-Enhancement Science for the Success of Society. With a keen interest in exploring the untapped potential of the human mind, Dr. Lowemann has dedicated his career to pushing the boundaries of human capabilities and understanding.
Armed with a Master of Science degree and a Ph.D. in his field, Dr. Lowemann has consistently been at the forefront of research and innovation, delving into ways to optimize human performance, cognition, and overall well-being. His work at the Institute revolves around a profound commitment to harnessing cutting-edge science and technology to help individuals lead more fulfilling and intelligent lives.
Dr. Lowemann’s influence extends to the educational platform BetterSmarter.me, where he shares his insights, findings, and personal development strategies with a broader audience. His ongoing mission is shaping the way we perceive and leverage the vast capacities of the human mind, offering invaluable contributions to society’s overall success and collective well-being.