Imagine trying to predict the outcome of a road trip just by looking at the car’s dashboard. That’s exactly what researchers did with a type of cancer called esophageal squamous cell carcinoma (ESCC). They used computed tomography (CT) images of patients to extract a set of high-tech features known as radiomics signature. This signature, combined with traditional staging and clinical information, formed a powerful predictive model. The results were impressive, with an accuracy rate of 87.8% in the training group and 85.7% in the validation group. By combining the radiomics signature with TNM staging, the model outperformed both factors alone, significantly improving survival predictions. These findings pave the way for more tailored treatment strategies for ESCC patients. If you’re curious about how they cracked this code, dive into the fascinating research behind it!
Cracking the Code of Esophageal Cancer Survival with CT Scans
To construct a prognostic model for preoperative prediction on computed tomography (CT) images of esophageal squamous cell carcinoma (ESCC), we created radiomics signature with high throughput radiomics features extracted from CT images of 272 patients (204 in training and 68 in validation cohort). Multivariable logistic regression was applied to build the radiomics signature and the predictive nomogram model, which was composed of radiomics signature, traditional TNM stage, and clinical features. A total of 21 radiomics features were selected from 954 to build a radiomics signature which was significantly associated with progression-free survival (p < 0.001). The area under the curve of performance was 0.878 (95% CI: 0.831–0.924) for the training cohort and 0.857 (95% CI: 0.767–0.947) for the validation cohort. The radscore of signatures’ combination showed significant discrimination for survival status. Radiomics nomogram combined radscore with TNM staging and showed considerable improvement over TNM staging alone in the training cohort (C-index, 0.770 vs. 0.603; p < 0.05), and it is the same with clinical data (C-index, 0.792 vs. 0.680; p < 0.05), which were confirmed in the validation cohort. Decision curve analysis showed that the model would receive a benefit when the threshold probability was between 0 and 0.9. Collectively, multiparametric CT-based radiomics nomograms provided improved prognostic ability in ESCC.
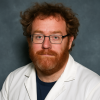
Dr. David Lowemann, M.Sc, Ph.D., is a co-founder of the Institute for the Future of Human Potential, where he leads the charge in pioneering Self-Enhancement Science for the Success of Society. With a keen interest in exploring the untapped potential of the human mind, Dr. Lowemann has dedicated his career to pushing the boundaries of human capabilities and understanding.
Armed with a Master of Science degree and a Ph.D. in his field, Dr. Lowemann has consistently been at the forefront of research and innovation, delving into ways to optimize human performance, cognition, and overall well-being. His work at the Institute revolves around a profound commitment to harnessing cutting-edge science and technology to help individuals lead more fulfilling and intelligent lives.
Dr. Lowemann’s influence extends to the educational platform BetterSmarter.me, where he shares his insights, findings, and personal development strategies with a broader audience. His ongoing mission is shaping the way we perceive and leverage the vast capacities of the human mind, offering invaluable contributions to society’s overall success and collective well-being.