Major Depressive Disorder (MDD) is like a fog that obscures people’s happiness, affecting their quality of life. Just as identifying shapes within a dense fog can be tedious and time-consuming, manually identifying MDD from brain structural magnetic resonance imaging (sMRI) images is laborious due to the lack of clear indicators. Scientists have developed automated methods using deep learning, but most are limited to 2D images, resulting in subpar performance. Additionally, the heterogeneous nature of MDD presents unique challenges when identifying the disorder based on brain sMRI images. That’s where the 3D FRN-ResNet comes in! This cutting-edge framework leverages 3D-ResNet to extract features and reconstruct them based on feature maps, capturing spatial details and location information. By solving the feature map reconstruction problem seamlessly, the model achieves outstanding performance. Researchers evaluated the framework using a private sMRI dataset of MDD patients and yielded impressive results that surpass other methods. To uncover more about this groundbreaking research, dive into the full article now!
Major Depressive Disorder (MDD) is the most prevalent psychiatric disorder, seriously affecting people’s quality of life. Manually identifying MDD from structural magnetic resonance imaging (sMRI) images is laborious and time-consuming due to the lack of clear physiological indicators. With the development of deep learning, many automated identification methods have been developed, but most of them stay in 2D images, resulting in poor performance. In addition, the heterogeneity of MDD also results in slightly different changes reflected in patients’ brain imaging, which constitutes a barrier to the study of MDD identification based on brain sMRI images. We propose an automated MDD identification framework in sMRI data (3D FRN-ResNet) to comprehensively address these challenges, which uses 3D-ResNet to extract features and reconstruct them based on feature maps. Notably, the 3D FRN-ResNet fully exploits the interlayer structure information in 3D sMRI data and preserves most of the spatial details as well as the location information when converting the extracted features into vectors. Furthermore, our model solves the feature map reconstruction problem in closed form to produce a straightforward and efficient classifier and dramatically improves model performance. We evaluate our framework on a private brain sMRI dataset of MDD patients. Experimental results show that the proposed model exhibits promising performance and outperforms the typical other methods, achieving the accuracy, recall, precision, and F1 values of 0.86776, 0.84237, 0.85333, and 0.84781, respectively.
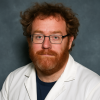
Dr. David Lowemann, M.Sc, Ph.D., is a co-founder of the Institute for the Future of Human Potential, where he leads the charge in pioneering Self-Enhancement Science for the Success of Society. With a keen interest in exploring the untapped potential of the human mind, Dr. Lowemann has dedicated his career to pushing the boundaries of human capabilities and understanding.
Armed with a Master of Science degree and a Ph.D. in his field, Dr. Lowemann has consistently been at the forefront of research and innovation, delving into ways to optimize human performance, cognition, and overall well-being. His work at the Institute revolves around a profound commitment to harnessing cutting-edge science and technology to help individuals lead more fulfilling and intelligent lives.
Dr. Lowemann’s influence extends to the educational platform BetterSmarter.me, where he shares his insights, findings, and personal development strategies with a broader audience. His ongoing mission is shaping the way we perceive and leverage the vast capacities of the human mind, offering invaluable contributions to society’s overall success and collective well-being.